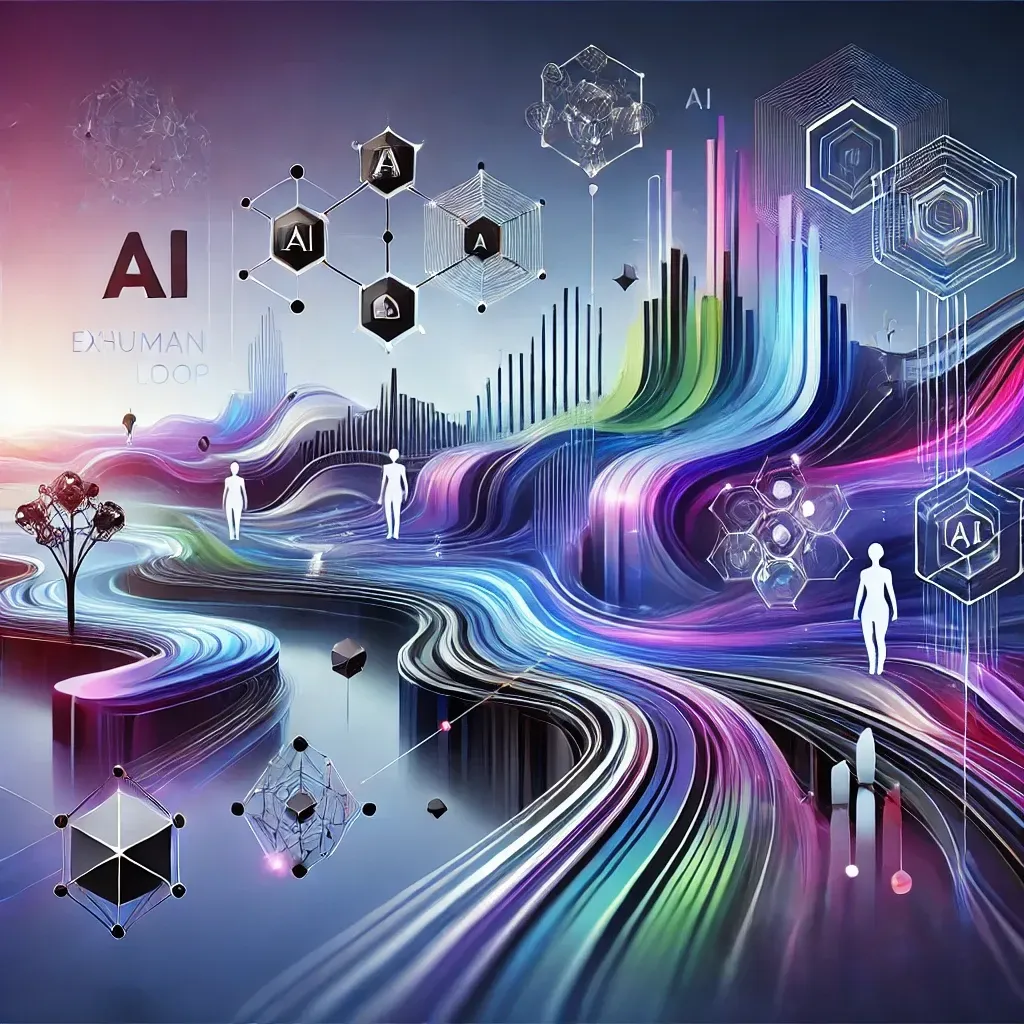
AI Automation Revolution: 5 Game-Changing Trends to Watch in 2024
As the realm of AI automation continues to expand, emerging trends are set to redefine industries and enhance operational efficiencies. In this blog, we’ll explore five pivotal research areas in AI-powered workflows that haven’t been widely discussed in previous analyses. Each section offers a detailed overview of these trends, including general insights, innovations, challenges, case studies, and sector-specific applications, providing a comprehensive understanding.
1. Adversarial Training for AI Models
General Insights
In the high-stakes worlds of healthcare and finance, adversarial training is rapidly becoming essential. This approach trains AI models to withstand adversarial attacks—intentionally deceptive inputs
designed to trick models into erroneous outputs. A recent study underscores its importance, demonstrating a 50% enhancement in model robustness through adversarial training (arXiv, 2024). The core idea is to anticipate possible manipulations by simulating potential adversarial scenarios during the model's training phase.
Innovations
The innovative use of Generative Adversarial Networks (GANs) and Adversarial Autoencoders (AAEs) represents a significant leap forward. These technologies enable AI systems to recognise and defend against sophisticated attacks, thereby fortifying their reliability. For instance, GANs can generate adversarial examples to test and reinforce model robustness, much like a digital sparring partner preparing an athlete for real-world competition.
Challenges
Despite its promise, adversarial training presents challenges, primarily its resource-intensive nature. Developing these robust systems requires significant computational power and specialised knowledge, posing hurdles for widespread adoption. Furthermore, there’s a pressing need to evaluate how these methods can be generalised across diverse applications.
Case Studies
A noteworthy example from Nature Medicine highlights adversarial training's role in strengthening AI diagnostic tools, enhancing their reliability (October 2024). In finance, as reported in the Financial Times, this training methodology has been pivotal in safeguarding AI systems against fraud and financial manipulation.
Sector-Specific Insights
In healthcare, this approach improves diagnostic precision, ensuring medical tools are resilient against data tampering, which could lead to misdiagnoses. Meanwhile, in finance, adversarial training fortifies the integrity of trading algorithms, enhancing trust and security in automated trading systems.
2. Explainable Graph Neural Networks (GNNs)
General Insights
Graph Neural Networks (GNNs) are increasingly utilised to handle complex, graph-structured data, crucial for applications like social network analysis and drug discovery. Explainable GNNs are emerging as a vital tool for enhancing the transparency of AI systems, enabling stakeholders to understand decision-making processes.
Innovations
Technological advances such as Graph Attention Networks (GATs) and Graph Convolutional Networks (GCNs) are paving the way. These models prioritise explainability by providing insights into how inputs influence outputs, akin to tracing the thought process behind a decision.
Challenges
The balance between interpretability and accuracy is a significant hurdle. While explainable models increase trust and understanding, they often sacrifice predictive performance. The field is still nascent, with ongoing research required to address these challenges.
Case Studies
A study highlighted in arXiv demonstrated improved interpretability in recommendation systems using explainable GNNs, enhancing user trust and engagement (October 2024). Another study in Manufacturing Technology showed how these networks could enhance predictive maintenance systems by making AI predictions more understandable.
Sector-Specific Insights
In healthcare, explainable GNNs can demystify AI diagnostic tools, leading to better patient outcomes by involving clinicians in the decision process. In finance, these networks enhance the transparency of trading systems, reducing the risks associated with opaque AI-driven decisions.
3. Autonomous Data Quality Management
General Insights
Autonomous data quality management leverages AI to ensure data is accurate, complete, and consistent. This approach can improve data quality by up to 90% (Data Science Journal, 2024), which is vital for sectors reliant on large datasets.
Innovations
Machine learning-based data validation and normalisation are revolutionising how organisations manage data quality. These methods minimise human intervention, enabling continuous quality assurance, much like self-regulating ecosystems.
Challenges
The complexity of integrating autonomous data quality management across heterogeneous data environments remains a challenge. Effective solutions require adaptable algorithms capable of learning from diverse data types and sources.
Case Studies
In healthcare, a study in Nature Medicine showed how improved data quality management led to more accurate AI diagnostics. In manufacturing, research in Manufacturing Technology demonstrated how enhanced data quality boosted the efficiency of predictive maintenance systems.
Sector-Specific Insights
In healthcare, reliable data leads to more trustworthy diagnostic tools, while in finance, high-quality data underpins the integrity of trading algorithms, reducing the risk of errors and enhancing decision-making accuracy.
4. Human-in-the-Loop (HITL) AI
General Insights
Human-in-the-Loop (HITL) AI integrates human oversight into AI systems, crucial in sectors where nuanced judgement is indispensable, such as healthcare and finance. By incorporating human feedback, AI models can continually refine their decision-making processes.
Innovations
Advancements in active learning and human-in-the-loop machine learning enable ongoing model improvements through expert feedback. This iterative learning process is similar to a teacher providing guidance and corrections to students, enhancing their learning curve.
Challenges
The availability and expertise of humans to provide effective oversight remain limiting factors. Solutions that scale human involvement efficiently are still under exploration, necessitating further research.
Case Studies
Research in Nature Medicine illustrated how HITL AI improved diagnostic accuracy by incorporating expert feedback. In manufacturing, a study showed enhanced predictive maintenance through expert input, as documented in Manufacturing Technology.
Sector-Specific Insights
In healthcare, HITL AI allows for more accurate diagnoses by integrating clinical expertise, while in finance, it enhances trading system security by combining human judgement with algorithmic precision.
5. AI-Powered Digital Twinning
General Insights
AI-powered digital twinning involves creating virtual models of physical systems to simulate and predict behaviours, crucial for optimising operations in sectors like manufacturing and energy management.
Innovations
Machine learning-based simulations and predictive analytics enhance digital twins, making them more reliable for strategic decision-making. These advancements are akin to using a flight simulator for pilots to practice and refine skills before real-world application.
Challenges
The complexity and volume of data required for digital twins pose significant challenges. Ensuring accurate and reliable simulations demands sophisticated data processing and model management.
Case Studies
A study in Manufacturing Technology highlighted how digital twins optimised industrial processes, while research in the Energy Management Journal showed improvements in predictive maintenance systems.
Sector-Specific Insights
In manufacturing, digital twins streamline operations, reducing costs and downtime. In energy management, they optimise maintenance schedules, resulting in significant energy savings and operational efficiency.
Conclusion
The trends discussed in this blog underscore the transformative potential of AI automation across various industries. By embracing adversarial training, explainable GNNs, autonomous data quality management, HITL AI, and AI-powered digital twinning, businesses can navigate the complexities of AI integration and harness these technologies for greater operational efficiency and innovation. Companies seeking to leverage these advancements should explore partnerships with AI automation and web development services to remain competitive in this rapidly evolving landscape.
References
arXiv, October 2024
Nature Medicine, October 2024
Financial Times, October 2024
Data Science Journal, October 2024
Manufacturing Technology, October 2024
Energy Management Journal, October 2024