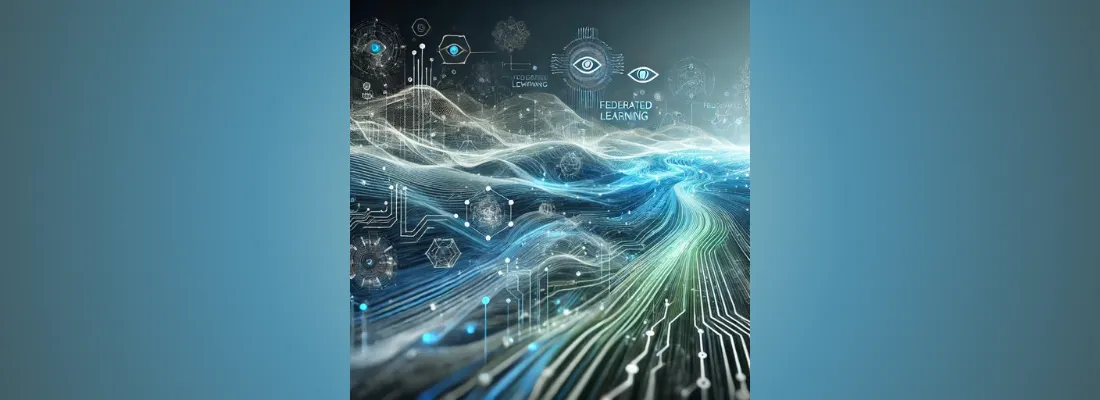
Revolutionary AI Automation Trends Set to Transform Industries
As the landscape of AI automation and web development continues to evolve, new trends are emerging that promise to reshape industries and enhance operational efficiencies. In this blog, we explore five key trends that have not been covered in previous discussions, providing insights into their innovations, challenges, and sector-specific applications.
1. Federated Learning
General Insights
Federated learning is a decentralized machine learning approach that enables multiple parties to collaboratively train models while keeping their data local. This method is particularly valuable in sectors where data privacy is paramount, such as healthcare and finance. By avoiding the centralization of data, federated learning reduces the risk of data breaches and ensures compliance with stringent data protection regulations.
Recent advancements in federated learning include the development of new algorithms that enhance model accuracy and efficiency. Techniques such as secure multi-party computation and differential privacy are being integrated to bolster data security during the training process. These innovations allow organizations to benefit from collaborative learning while maintaining the privacy and security of their data.
Despite its potential, federated learning faces significant challenges. Ensuring the security of data during transmission and addressing the non-IID (independent and identically distributed) nature of data across different parties can complicate model training. These issues can lead to reduced model performance and reliability.
Case Studies
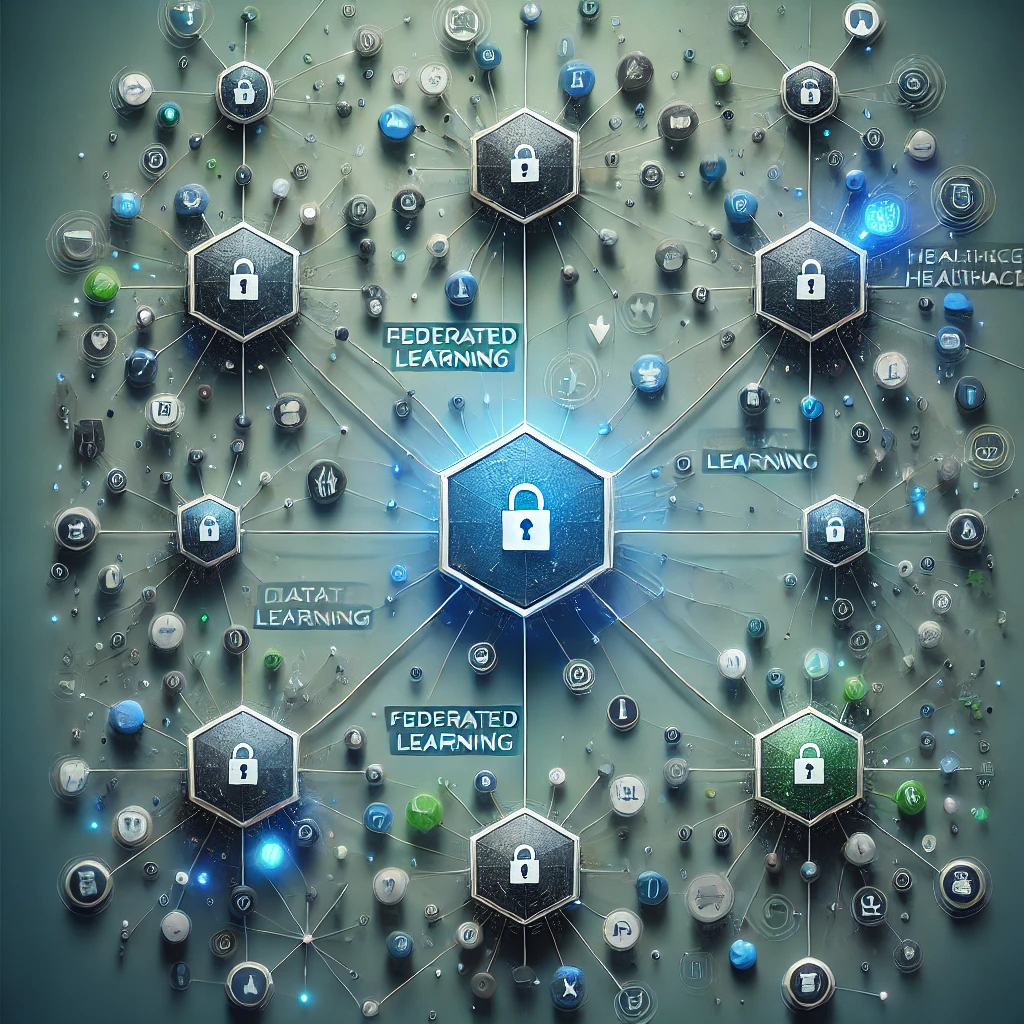
Google: The tech giant has pioneered federated learning through its TensorFlow Federated framework, enabling various industries to train models without compromising sensitive data.
Healthcare: Hospitals are utilizing federated learning to collaboratively develop predictive models for patient outcomes, allowing them to improve care without sharing patient data.
Sector-Specific Insights
Healthcare: Federated learning can facilitate the development of predictive analytics tools that enhance patient care while maintaining confidentiality.
Finance: Financial institutions can leverage federated learning for credit risk assessments, enabling them to build robust models without exposing customer data.
2. Graph Neural Networks (GNNs) for Recommendation Systems
General Insights
Graph Neural Networks (GNNs) are specialized neural networks designed to process graph-structured data. They are increasingly being applied in recommendation systems to enhance accuracy and personalization. Unlike traditional neural networks, which operate on flat data, GNNs work with data points connected by relationships, much like social networks or molecular structures.
Recent innovations in GNNs include the introduction of graph attention networks and graph convolutional networks, which allow for a more nuanced understanding of user interactions and preferences. These models can capture complex relationships and provide more precise recommendations.
One of the primary challenges in implementing GNNs is managing large-scale graph data, which can be computationally intensive. Additionally, ensuring the interpretability of GNN models remains a significant hurdle, as stakeholders need to understand how recommendations are generated.
Case Studies
Pinterest: The platform has successfully integrated GNNs into its recommendation engine, resulting in a 20% improvement in recommendation accuracy.
E-commerce: Companies are employing GNNs to analyze user behavior and preferences, leading to more tailored product recommendations.
Sector-Specific Insights
E-commerce: GNNs can significantly enhance user experience by providing personalized product suggestions based on complex user interaction data.
Social Media: Platforms can utilize GNNs to recommend content that aligns with user interests, improving engagement and retention.
3. Explainable Reinforcement Learning (XRL)
General Insights
Explainable Reinforcement Learning (XRL) focuses on making the decision-making processes of AI agents transparent. This is crucial in applications where trust and accountability are essential, such as finance and healthcare. XRL seeks to demystify how AI systems arrive at their decisions, making the technology more accessible and trustworthy.
Innovative techniques in XRL include model-based reinforcement learning and inverse reinforcement learning, which provide clearer insights into how decisions are made by AI systems. These approaches aim to create models that not only perform well but also offer understandable rationales for their actions.
XRL faces challenges related to the complexity of decision-making processes, which can be difficult to interpret. Ensuring that explanations are understandable to non-experts is also a significant barrier.
Case Studies
Google: The company has developed the XRL-Kit framework, which aids in understanding AI decision-making processes in various applications.
Robotics: XRL is being utilized in robotics to clarify the decision-making processes of autonomous systems, enhancing user trust.
Sector-Specific Insights
Robotics: XRL can improve the safety and reliability of robotic systems by providing insights into their decision-making processes.
Finance: Financial institutions can employ XRL to explain trading decisions made by AI systems, fostering trust among stakeholders.
4. Digital Twins for Predictive Maintenance
General Insights
Digital twins are virtual representations of physical systems that can be used to monitor and predict equipment performance. This technology is gaining traction in industries where equipment reliability is critical. By creating a digital replica of physical assets, organizations can simulate and analyze their operations in real-time.
Recent advancements in digital twin technology include the integration of machine learning algorithms and IoT data, which enhance the predictive capabilities of these virtual models. These innovations enable more accurate predictions of equipment failures and maintenance needs, reducing downtime and costs.
One of the main challenges in implementing digital twins is ensuring the accuracy of the virtual models. Additionally, managing the complexity of real-world systems can complicate the development of effective digital twins.
Case Studies
GE Appliances: The company has developed a digital twin platform that predicts equipment failures, resulting in a 20% reduction in downtime.
Manufacturing: Digital twins are being used to optimize production processes by predicting equipment failures before they occur.
Sector-Specific Insights
Manufacturing: Digital twins can significantly enhance operational efficiency by predicting equipment failures and facilitating proactive maintenance.
Energy: In power plants, digital twins can help in monitoring equipment health, thereby reducing operational risks and costs.
5. Conversational AI for Mental Health
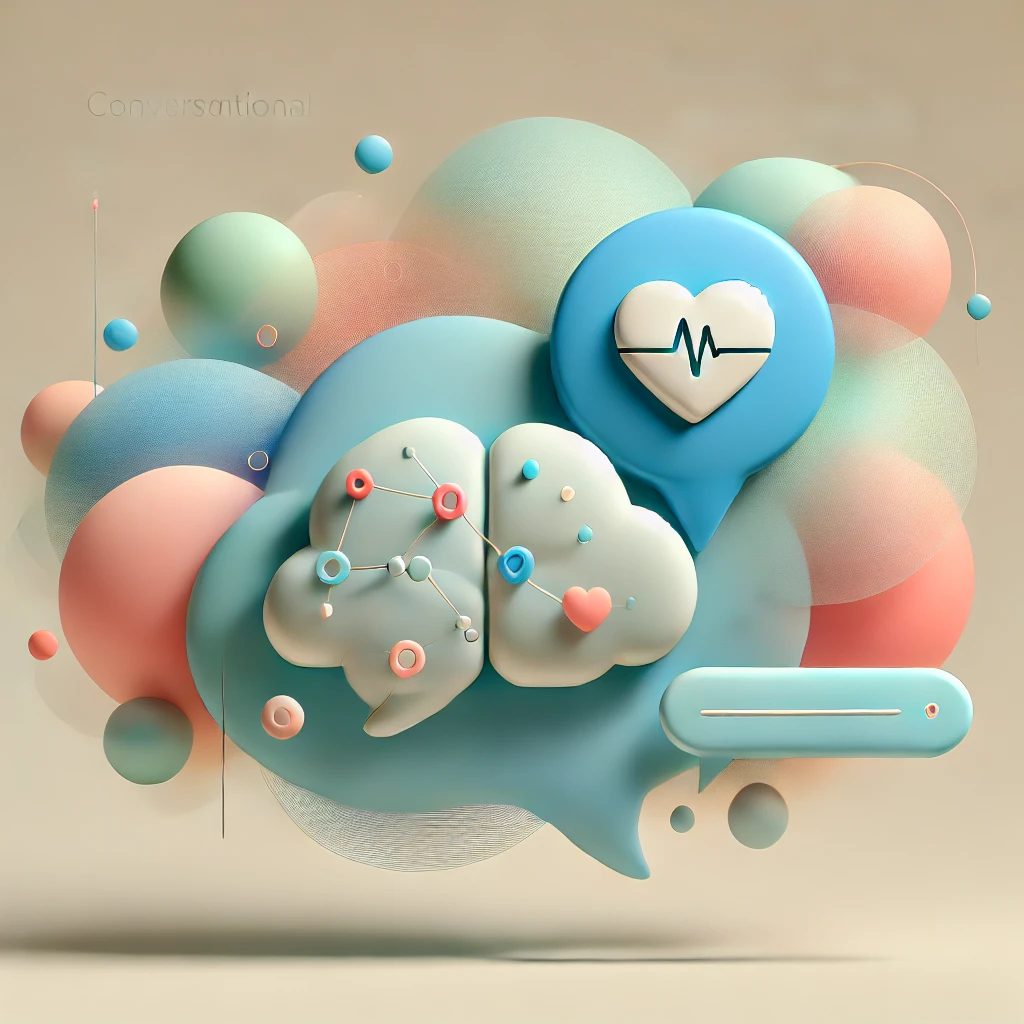
General Insights
Conversational AI utilizes natural language processing and machine learning to provide mental health support through chat interfaces. This technology is becoming increasingly important in delivering accessible mental health care. By simulating human conversation, conversational AI can offer support and guidance to users in a scalable and consistent manner.
Innovations in conversational AI for mental health include the integration of emotional intelligence and empathy, enabling more human-like interactions that can enhance user experience. These advancements allow AI to recognize and respond to users' emotional states, providing more effective support.
Challenges in this field include ensuring the accuracy and effectiveness of therapeutic interventions delivered by AI. Additionally, handling sensitive personal data responsibly is paramount.
Case Studies
Woebot: This platform leverages conversational AI to provide mental health support, using evidence-based techniques to assist users.
Healthcare: Various healthcare providers are incorporating conversational AI to offer mental health support, making therapy more accessible to patients.
Sector-Specific Insights
Healthcare: Conversational AI can bridge gaps in mental health services, providing support to individuals who may not have access to traditional therapy.
Education: Schools are beginning to use conversational AI to offer mental health resources to students, promoting well-being in educational environments.
Conclusion
The trends outlined above represent significant advancements in AI automation and web development that are set to transform various industries. By understanding these innovations, challenges, and sector-specific applications, professionals can harness the potential of these technologies to drive growth and improve operational efficiencies.
As businesses prepare for these advancements, it is crucial to explore how these AI trends can be integrated into their operations. Partnering with an AI automationand web development agency can provide the expertise needed to leverage these cutting-edge technologies effectively.
Bibliography
Google AI Blog (2023). Federated Learning: Opportunities and Challenges.
Pinterest Engineering Blog (2023). Enhancing Recommendations with Graph Neural Networks.
Google Research (2023). Explainable Reinforcement Learning: Insights and Applications.
GE Appliances (2023). The Impact of Digital Twins on Predictive Maintenance.
Woebot Health (2023). Conversational AI for Mental Health: Innovations and Insights.