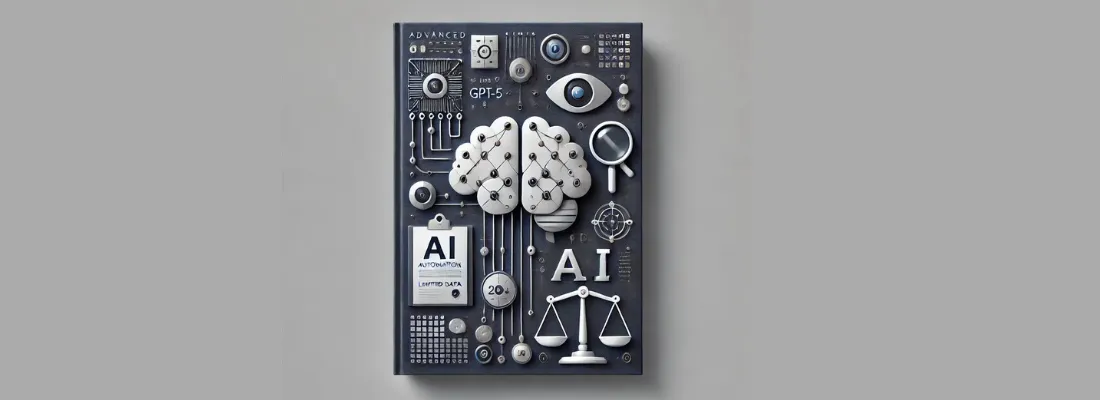
Revolutionising the Future: Top AI Automation Trends in Web Development
As the landscape of AI automation and web development continues to evolve, new trends are emerging that promise to reshape industries and redefine the way we interact with technology. In this blog, we’ll focus on five key trends that have not been previously discussed in earlier posts, providing comprehensive insights into their innovations, challenges, and potential applications.
1. New AI Models and Engines: The Rise of GPT-5 and Claude
General Insights
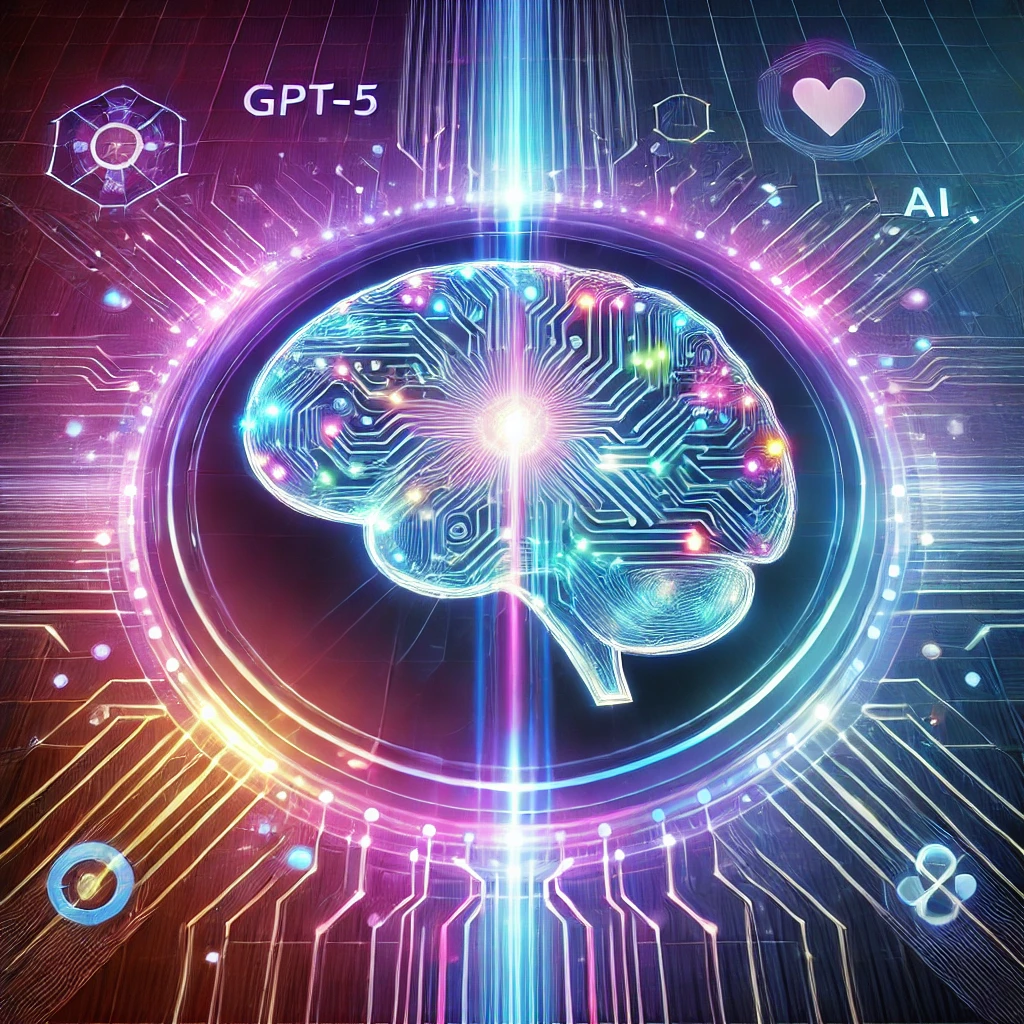
The development of advanced AI models such as GPT-5 and Claude represents a significant leap in natural language processing (NLP). These models are designed to enhance language understanding and generation, paving the way for more intuitive interactions between humans and machines. With their capabilities, sectors like customer service, marketing, and healthcare stand to benefit immensely.
Innovations
GPT-5 and Claude introduce groundbreaking capabilities in understanding human emotions and context, allowing for more empathetic and personalised interactions. Imagine a customer service chatbot not only responding to inquiries but also gauging the emotional state of the customer to adjust its tone and solutions accordingly. This could revolutionise customer service by providing more satisfying user experiences.
Challenges
Despite their advancements, these models are not without challenges. Bias and stereotyping are significant concerns, as these models may inadvertently reflect biases present in their training data. Ensuring these AI systems are fair and unbiased is crucial for their responsible deployment. Businesses must prioritise ethical considerations to avoid reinforcing negative stereotypes and perpetuating inequities.
Case Studies
IBM's Watson serves as a pertinent example, demonstrating successful emotion-aware AI in customer service and healthcare. By harnessing similar models, companies can enhance their service offerings, leading to improved patient engagement through personalised communication in healthcare settings.
Sector-Specific Insights
In healthcare, GPT-5 and Claude could facilitate the development of chatbots that assist patients with medical inquiries, providing tailored responses based on individual needs. In customer service, these models can create more engaging and effective support systems, ultimately enhancing customer loyalty. These advancements not only improve service delivery but also position businesses as leaders in AI-driven customer engagement.
2. Machine Learning Algorithms: Learning from Limited Data
General Insights
A recent machine learning algorithm developed by researchers at Stanford University showcases an impressive ability to learn from limited data, a feature that is particularly valuable in real-world scenarios where data is scarce. This advancement could revolutionise how machine learning is applied across various industries by making it feasible even in data-sparse environments.
Innovations
The algorithm's ability to function effectively with minimal data marks a significant innovation in machine learning. Traditional models often require vast datasets to learn effectively, but this new approach allows for robust models that can adapt to new tasks with limited data. This is especially useful in sectors like healthcare, where data collection can be challenging due to privacy concerns.
Challenges
A notable challenge is overfitting, where a model becomes overly tailored to its training data and struggles to generalise to new situations. Ensuring the algorithm maintains predictive power across diverse datasets will be critical for its success. Techniques such as cross-validation and regularisation may be employed to mitigate these risks.
Case Studies
Google's use of similar machine learning techniques in developing algorithms capable of learning from limited data, particularly in image recognition and NLP, highlights the versatility and potential for widespread adoption of these algorithms. This ability allows companies to create models that perform well even when large datasets are unavailable.
Sector-Specific Insights
In finance, this algorithm could enhance predictive modelling, allowing institutions to identify risks and opportunities more effectively. In retail, it can refine recommendation systems, helping customers discover products that align with their preferences, thereby boosting sales and customer satisfaction. By employing these advanced algorithms, businesses can optimise operations and gain a competitive edge.
3. Computer Vision: Advancements in Visual Data Analytics
General Insights
Recent advancements in computer vision technology are set to transform visual data analytics, enabling more sophisticated systems for image recognition, object detection, and tracking. These developments are particularly relevant in industries such as healthcare, finance, and retail, where accurate data interpretation is paramount.
Innovations
The innovations in computer vision enable systems to analyse visual data with unprecedented accuracy, enhancing decision-making processes and operational efficiencies. For example, retail businesses can use computer vision for real-time inventory management, reducing errors and improving customer service.
Challenges
Nevertheless, the technology faces challenges related to bias and stereotyping. Computer vision systems must be trained on diverse datasets to mitigate these risks and ensure fairness in their applications. Addressing these issues is crucial to prevent discriminatory practices and to foster trust among users.
Case Studies
Amazon's use of computer vision technology for product recognition and tracking exemplifies its effectiveness. The company's systems have streamlined inventory management and improved product recommendations, enhancing operational efficiency and customer experience.
Sector-Specific Insights
In healthcare, computer vision can aid in medical image analysis, supporting diagnostics and treatment planning. By providing precise image interpretations, these systems can improve patient outcomes. In retail, computer vision enhances customer experiences through personalised recommendations based on visual data analysis, driving engagement and sales. Businesses leveraging computer vision can significantly enhance their analytical capabilities and operational efficiencies.
4. Ethical Considerations: Emphasising AI Ethics
General Insights
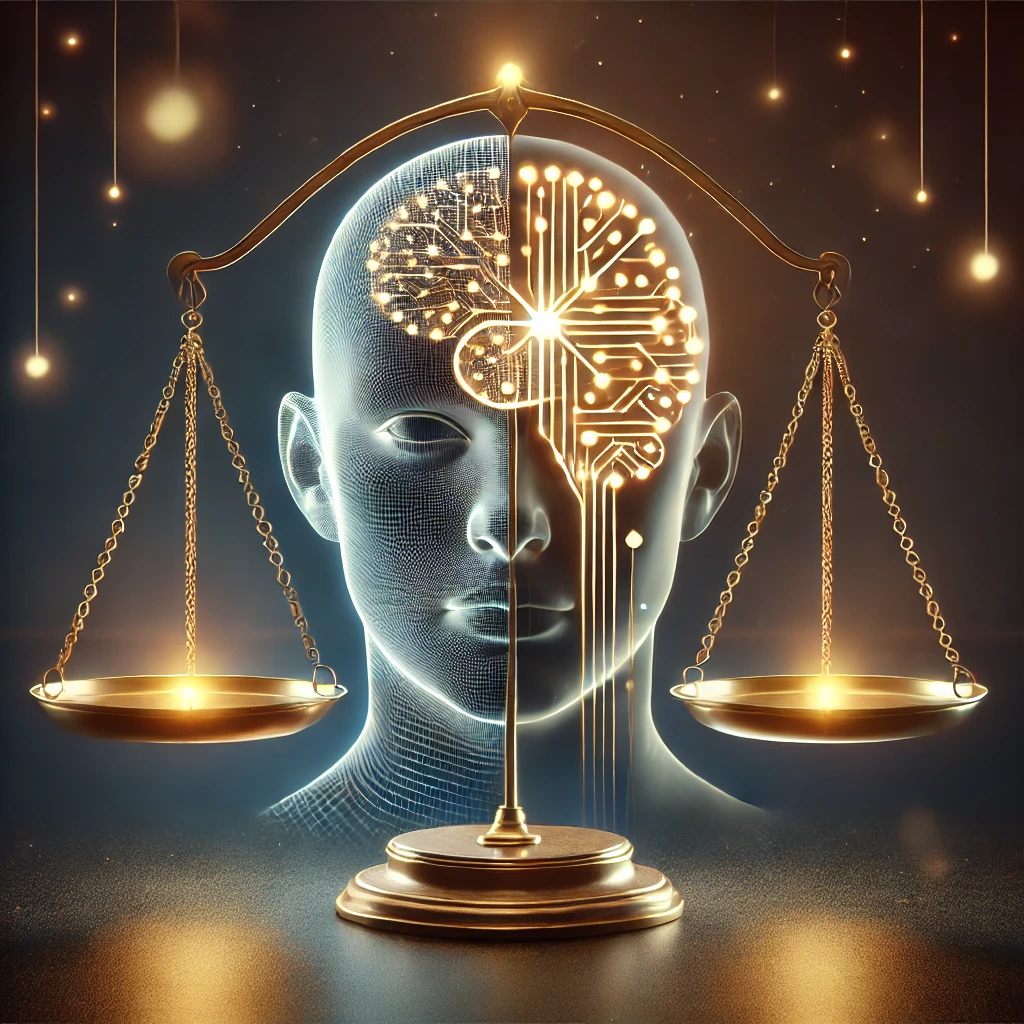
The growing concerns regarding AI systems perpetuating biases highlight the urgent need for a stronger focus on AI ethics. Researchers at Harvard University emphasise that ethical considerations must be integrated into AI technology development to prevent harmful outcomes and foster trust.
Innovations
The establishment of AI ethics frameworks is a significant innovation in the field. These frameworks guide developers in creating fair and unbiased AI systems, promoting accountability and transparency. By adhering to ethical standards, businesses can build trust with their customers and stakeholders.
Challenges
One primary challenge is the potential for bias to be inadvertently encoded within ethical guidelines themselves. Continuous evaluation and adaptation of these frameworks are necessary to ensure their effectiveness. Businesses must remain vigilant and proactive in addressing ethical concerns to maintain credibility and public trust.
Case Studies
Microsoft's development of a bias detection system showcases the practical application of AI ethics. This system has been employed across various applications, including NLP and image recognition, to promote fairness and accountability. Such initiatives demonstrate the potential of ethical AI to enhance business operations and foster equitable outcomes.
Sector-Specific Insights
In finance, AI ethics can help develop systems ensuring fairness in lending and investment decisions. By prioritising ethical considerations, financial institutions can avoid discriminatory practices and improve customer relations. In healthcare, ethical guidelines can guide AI tools development that prioritises patient welfare and equitable access to care, enhancing trust and patient outcomes. By integrating ethics into AI development, businesses can drive innovation while maintaining social responsibility.
5. Regulatory Trends: EU Regulations on AI
General Insights
The introduction of new regulations by the European Union focusing on transparency, accountability, and human oversight in AI development signifies a significant shift in the regulatory landscape. These regulations aim to ensure AI technologies are developed and deployed responsibly, protecting consumers and fostering innovation.
Innovations
The EU's regulatory framework introduces requirements for AI systems, emphasising transparency in decision-making processes. This approach encourages companies to adopt practices that enhance accountability and public trust in AI technologies. By aligning with these regulations, businesses can improve their competitive position in the global market.
Challenges
While these regulations aim to protect consumers and promote ethical AI, there is a risk of over-regulation potentially stifling innovation. Striking a balance between regulation and fostering technological advancement will be crucial for the industry's growth. Businesses must navigate this regulatory environment carefully to remain compliant and innovative.
Case Studies
Google's proactive approach to developing transparent AI systems illustrates how companies can align with regulatory expectations while maintaining innovation. By implementing measures that enhance accountability, businesses can navigate the evolving regulatory landscape effectively, ensuring compliance and continued growth.
Sector-Specific Insights
In finance, these regulations can lead to developing systems ensuring transparency in algorithmic trading and lending practices. By adhering to these standards, financial institutions can improve consumer confidence and market stability. In healthcare, regulations can promote accountability in AI-driven diagnostics and treatment recommendations, enhancing patient care and trust. Businesses that embrace these regulatory changes will be well-positioned to thrive in a rapidly evolving technological landscape.
Conclusion
The landscape of AI automation and web development is rapidly evolving, with new trends emerging that promise to reshape industries and improve the way we interact with technology. By focusing on innovations in AI models, machine learning algorithms, computer vision, ethical considerations, and regulatory trends, businesses can position themselves at the forefront of this transformative wave. As we move into 2024, understanding and adapting to these trends will be essential for maintaining competitiveness and driving innovation. Companies are encouraged to explore AI automation services to stay ahead of the curve and leverage these advancements for strategic growth.
Sources and References
Stanford University Research on Machine Learning Algorithms.
IBM Watson Case Study on Emotion-Aware AI.
Amazon's Implementation of Computer Vision Technology.
Harvard University Research on AI Ethics.
European Union AI Regulations Overview.