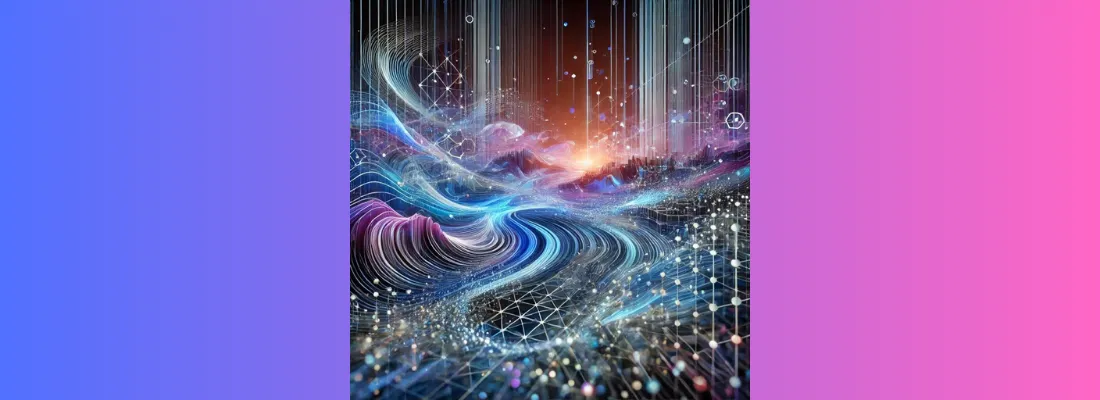
Revolutionizing Web Development: The Cutting-Edge Trends in AI Automation
Emerging Trends in AI Automation: A Fresh Perspective
As the landscape of AI automation and web development continues to evolve, new trends are emerging that promise to redefine how industries operate. In this blog, we will explore five innovative trends that have not been previously discussed, providing insights into their implications, innovations, challenges, and sector-specific applications. Our focus will be on Multimodal Learning, Cognitive Architectures, Explainable Planning, Swarm Intelligence, and Neuromorphic Computing.
1. Multimodal Learning
General Insights
Multimodal learning is an exciting field that enables AI systems to process and interpret various types of data—such as text, images, and audio—simultaneously. Picture an orchestra, where each instrument represents a different data type. Just as the conductor ensures these instruments produce harmonious music, multimodal learning integrates these data forms to create a comprehensive understanding. This capability is essential for applications requiring a holistic grasp of information, such as autonomous vehicles interpreting traffic signs, road conditions, and verbal commands all at once.
Innovations
Recent advancements include architectures like the Multimodal Transformer and the Multimodal Attention Mechanism. These innovations facilitate sophisticated interactions between different data modalities, enhancing the model's ability to derive insights from complex datasets. For instance, in sentiment analysis, multimodal learning combines video footage, audio tone, and text to accurately gauge public sentiment, which is invaluable for businesses monitoring brand reputation.
Challenges
The primary challenge lies in seamlessly integrating diverse data types while maintaining each modality's semantic integrity. Ensuring accurate interpretation and relationship of information from different sources is vital for effective learning and application.
Sector-Specific Insights
Healthcare: Multimodal learning can significantly enhance diagnostic accuracy by integrating medical imaging with patient history and genetic data, offering a comprehensive view that aids in precision medicine.
Finance: By analysing financial news alongside market data, AI can better predict stock movements and identify emerging trends, providing businesses with a strategic advantage.
Retail: Understanding customer behaviour through various data forms can lead to more personalised marketing strategies, increasing customer satisfaction and loyalty.
2. Cognitive Architectures
General Insights
Cognitive architectures aim to replicate human cognitive processes within AI systems, enabling more efficient decision-making. Think of these architectures as the blueprint of human thought processes, facilitating a deeper understanding of complex tasks. This trend is gaining traction in robotics, natural language processing, and computer vision, allowing AI systems to make decisions akin to human reasoning.
Innovations
Innovations in this area include the Cognitive Architecture for Robotics and the Neural Cognitive Architecture, which enable systems to adapt and perform complex tasks more intelligently. For example, in robotics, these architectures allow machines to autonomously adjust to changing environments, improving operational efficiency in dynamic settings like warehouses.
Challenges
Integrating multiple cognitive components while preserving their semantic meanings poses a significant challenge. It is crucial to ensure these components work harmoniously for the overall effectiveness of cognitive architectures.
Sector-Specific Insights
Manufacturing: Cognitive architectures can optimise production lines by enhancing robotic systems' adaptability, thereby reducing downtime and increasing productivity.
Healthcare: By analysing vast amounts of patient data, these architectures can assist in making more informed clinical decisions, leading to better patient outcomes.
Finance: They improve risk assessment models by effectively analysing market trends and historical data, offering better financial strategies.
3. Explainable Planning
General Insights
Explainable planning focuses on developing AI systems that can articulate their decision-making processes, akin to a GPS explaining its chosen route. This transparency is essential for fostering trust and accountability in AI applications, particularly in critical sectors like healthcare and finance.
Innovations
New algorithms such as the Explainable Planning Algorithm and the Transparent Planning Framework have been developed to enhance the clarity of AI decision-making processes. These tools ensure that AI's decisions are understandable, thus fostering trust among users and stakeholders.
Challenges
A significant hurdle is creating systems that can provide clear, concise explanations of their planning processes. Ensuring these explanations are accessible to non-experts is vital for broader acceptance.
Sector-Specific Insights
Manufacturing: Explainable planning can enhance transparency in robotic operations, improving collaboration between humans and machines, thus streamlining workflows.
Healthcare: By elucidating the reasoning behind treatment recommendations, AI can build trust with patients and healthcare providers, leading to more accepted treatment plans.
Finance: Explainable planning aids in regulatory compliance by providing clear justifications for financial decisions made by AI systems, enhancing transparency and reducing the risk of disputes.
4. Swarm Intelligence
General Insights
Swarm intelligence leverages the collective behaviour of decentralised systems, similar to how birds flock together to navigate complex terrains. This approach can lead to innovative solutions across various fields, from optimising logistics to managing crowds.
Innovations
Developments include the Swarm Intelligence Algorithm and the Flocking Algorithm, which mimic natural swarming behaviours to improve decision-making processes in AI systems. For example, these algorithms can enhance disaster response strategies by coordinating autonomous drones to deliver supplies efficiently.
Challenges
A key challenge is developing real-time analysis capabilities to understand and predict the behaviour of complex systems effectively. This requires robust computational power and sophisticated algorithms.
Sector-Specific Insights
Manufacturing: Swarm intelligence can optimise production processes by coordinating multiple robotic units, leading to enhanced efficiency and reduced operational costs.
Healthcare: By analysing patient data through swarm intelligence, healthcare providers can develop better treatment strategies tailored to individual needs, improving patient outcomes.
Finance: This approach can enhance market analysis by simulating the behaviour of various market participants, leading to more accurate predictions and better investment strategies.
5. Neuromorphic Computing
General Insights
Neuromorphic computing aims to design AI systems that emulate the human brain's structure and function. Imagine these systems as super-efficient brains capable of learning and adapting much like humans. This trend promises enhanced processing efficiency and adaptability in AI applications.
Innovations
Recent advancements include the Neuromorphic Computing Architecture and the Spiking Neural Network Algorithm, which allow for more efficient data processing and learning. These innovations are crucial for real-time data analysis and decision-making, providing businesses with a competitive edge in fast-paced markets.
Challenges
The complexity of accurately mimicking the brain's architecture and functionality remains a significant challenge, necessitating ongoing research and development to fully realise its potential.
Sector-Specific Insights
Healthcare: Neuromorphic systems can analyse medical data more efficiently, leading to faster diagnoses and more effective treatment plans, thus improving healthcare delivery.
Finance: These systems can enhance predictive analytics by processing vast amounts of financial data in real time, aiding in timely investment decisions and risk management.
Retail: Neuromorphic computing can improve customer experience through personalised recommendations based on behaviour analysis, thereby increasing sales and customer satisfaction.
Conclusion
The trends outlined above—multimodal learning, cognitive architectures, explainable planning, swarm intelligence, and neuromorphic computing—represent the forefront of AI automation and web development research. By understanding and leveraging these innovations, industries can enhance their operational efficiency, improve decision-making processes, and foster greater trust in AI systems. As these technologies continue to evolve, they will undoubtedly play a pivotal role in shaping the future of various sectors. Businesses that embrace these advancements can position themselves as leaders in innovation, efficiency, and customer engagement.
As these AI technologies advance, businesses can prepare by investing in training, developing strategic AI integration plans, and collaborating with AI specialists to implement these systems effectively. Embracing these trends not only enhances current operations but also future-proofs businesses against the ever-evolving digital landscape. For companies looking to stay ahead, exploring AI automation services can be a crucial step in maintaining a competitive edge.
References
Nature Machine Intelligence. (2023). Multimodal Learning for Sentiment Analysis.
Science Robotics. (2023). Cognitive Architectures in Robotic Systems.
IEEE Robotics and Automation Letters. (2023). Explainable Planning in Robotics.
Science. (2023). Swarm Intelligence and Collective Behaviour Analysis.
Nature. (2023). Neuromorphic Computing for Image Recognition.
Meta Information
Meta Title: AI Automation for Web Development | Streamline Your Business
Meta Description: Discover the top AI automation tools for web development. Learn how AI workflows can transform your business and save costs. Read more here.