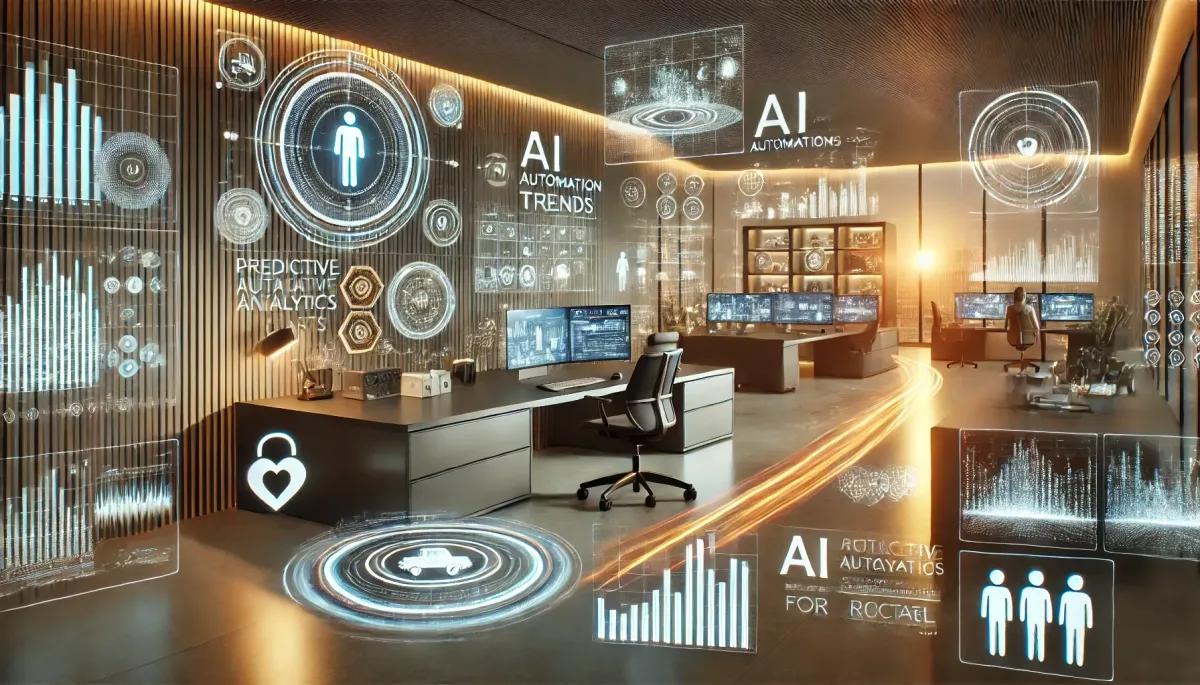
Unlocking the Future: Essential AI Trends for 2024
Unlocking the Future: Essential AI= Trends for 2024
Introduction
Artificial Intelligence (AI) is continually evolving, pushing the boundaries of what AI automation tools can achieve across various industries. As we move into 2024, it’s essential for businesses to stay informed about the latest trends to harness AI's full potential. In this blog, we will explore five emerging AI automation trends, including new AI models, machine learning algorithms, advancements in computer vision, autonomous data quality management, and AI-powered digital twinning. Each trend presents unique opportunities and challenges that could redefine operational efficiency and strategic decision-making in business.
1. New AI Models and Engines: The Rise of GPT-5
The development of GPT-5 marks a significant leap in natural language processing (NLP). This model excels in various linguistic tasks, such as language translation, text summarisation, and conversational AI, enhancing business communication and customer interaction. What sets GPT-5 apart is its ability to learn from limited data inputs, allowing it to adapt quickly to specific tasks with minimal training. This feature makes it a versatile tool for sectors that require rapid adaptability.
However, the computational demands of GPT-5 can pose challenges for many organisations. Deploying this technology necessitates considerable infrastructure investment to fully harness its capabilities. For instance, in the healthcare sector, GPT-5 can facilitate real-time language translation during telemedicine consultations, bridging language barriers between patients and healthcare providers. In finance, analysts utilise GPT-5 to distil complex reports and market trends, streamlining data interpretation and decision-making processes. In retail, chatbots powered by GPT-5 enhance customer service by providing immediate and accurate responses, thereby boosting customer satisfaction.
Overall, GPT-5's sector-specific applications—from streamlining medical transcription in healthcare to offering personalised recommendations in retail—illustrate its transformative potential. Businesses that are prepared to invest in the necessary infrastructure will find GPT-5 a valuable asset in driving customer engagement and operational efficiency.
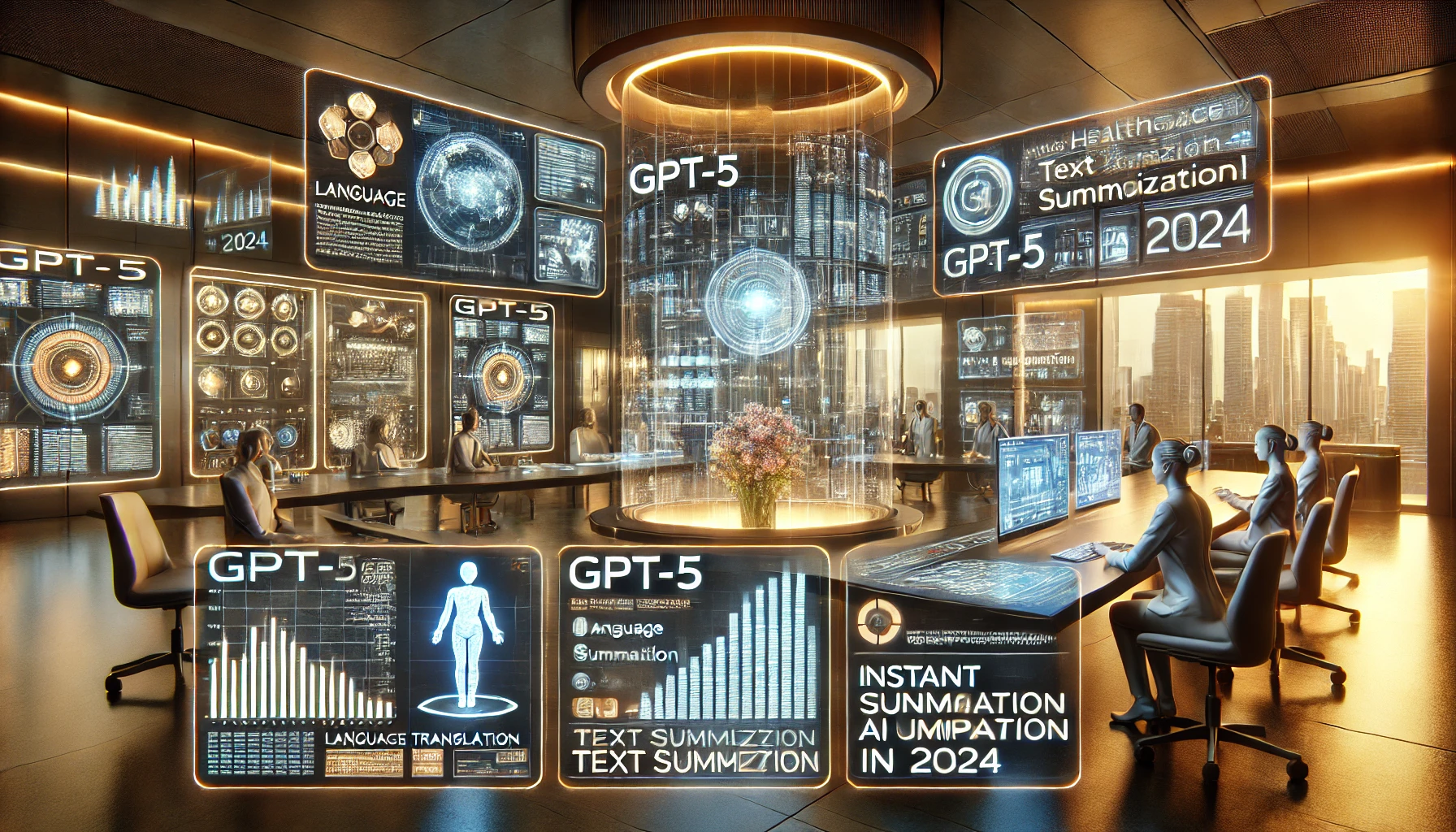
2. Machine Learning Algorithms: Learning from Limited Data
Recent advancements in machine learning, particularly in meta-learning and few-shot learning, enable systems to learn effectively from limited datasets. This capability is crucial for industries where data collection is challenging or expensive. These algorithms can adapt swiftly to new tasks with minimal examples, making them ideal for dynamic environments that require quick learning.
The primary challenge lies in ensuring these algorithms can generalise well across different tasks and environments, which necessitates ongoing research and development. For example, in medical diagnostics, these algorithms are applied to classify images, achieving high accuracy even with small training datasets. In natural language processing, few-shot learning enhances chatbots' ability to understand and respond to user queries efficiently.
In healthcare, these algorithms expedite diagnoses by analysing medical images with limited labelled data. In finance, they assist in predicting market trends using fewer historical data points, enabling timely investment decisions. In retail, personalisation engines leverage these algorithms to tailor recommendations based on minimal customer interaction history.
The ability of these algorithms to learn rapidly and effectively from limited data not only reduces costs but also enhances the responsiveness and accuracy of AI systems across various sectors, making them an invaluable tool for businesses aiming to optimise operations.
3. Computer Vision: Advancements in Visual Data Analytics
Computer vision has seen remarkable advancements, enabling machines to interpret and understand visual data with unprecedented accuracy. This technology is reshaping industries by providing insights that were previously unattainable. Innovations such as deep learning and transfer learning have significantly improved image recognition and object detection systems, allowing for real-time analysis of visual data.
One of the main challenges is ensuring that computer vision systems generalise well across different environments and tasks. For instance, in healthcare, computer vision analyses medical images for early disease detection, thereby improving patient outcomes. In finance, algorithms analyse visual data from trading floors to detect patterns and anomalies in real-time, aiding in faster decision-making. In retail, computer vision monitors customer behaviour in stores, optimising layout and inventory management.
By automating the analysis of visual data, businesses can enhance diagnostic accuracy in healthcare, facilitate fraud detection in finance, and improve customer experiences in retail. These advancements highlight the growing importance of computer vision in driving efficiency and innovation across diverse industries.
4. Autonomous Data Quality Management: Ensuring Data Integrity
In an era where data-driven decision-making is paramount, autonomous data quality management emerges as a crucial trend. This approach leverages AI to enhance the accuracy and consistency of data across organisations. Techniques such as machine learning and deep learning are employed to automatically clean, integrate, and validate data, ensuring high-quality datasets for analysis.
Adapting these systems to new data sources and environments remains a significant challenge, necessitating continuous improvement and monitoring. For instance, in the healthcare sector, AI-driven solutions are implemented to automate the cleaning of patient data, thereby enhancing treatment outcomes and research capabilities. In finance, autonomous data management ensures the integrity of financial data, reducing errors in reporting and compliance. In retail, it improves customer data quality, leading to better-targeted marketing strategies.
By ensuring data integrity, businesses can enhance decision-making processes and optimise operations. As data becomes increasingly central to business strategy, investing in autonomous data quality management will be crucial for maintaining a competitive advantage.
5. AI-Powered Digital Twinning: Optimising Operations
AI-powered digital twinning involves creating virtual models of physical systems to optimise operations. This trend is gaining traction as businesses seek to enhance efficiency and reduce costs. Advanced machine learning and simulation techniques allow for real-time analysis and optimisation of virtual models, improving decision-making and operational strategies.
The complexity of creating accurate digital twins and ensuring they reflect real-world conditions poses significant challenges. However, when implemented effectively, digital twins offer immense benefits. In manufacturing, companies use digital twins to simulate production processes, identify bottlenecks, and optimise workflows. In logistics, AI-driven models aid in route optimisation, reducing delivery times and costs. In energy management, digital twins simulate energy consumption patterns, enabling more efficient resource allocation.
By adopting digital twinning, businesses can streamline operations, enhance predictive maintenance, and improve resource management. This innovative approach to operational efficiency is set to become a cornerstone of industry strategy in the coming years.
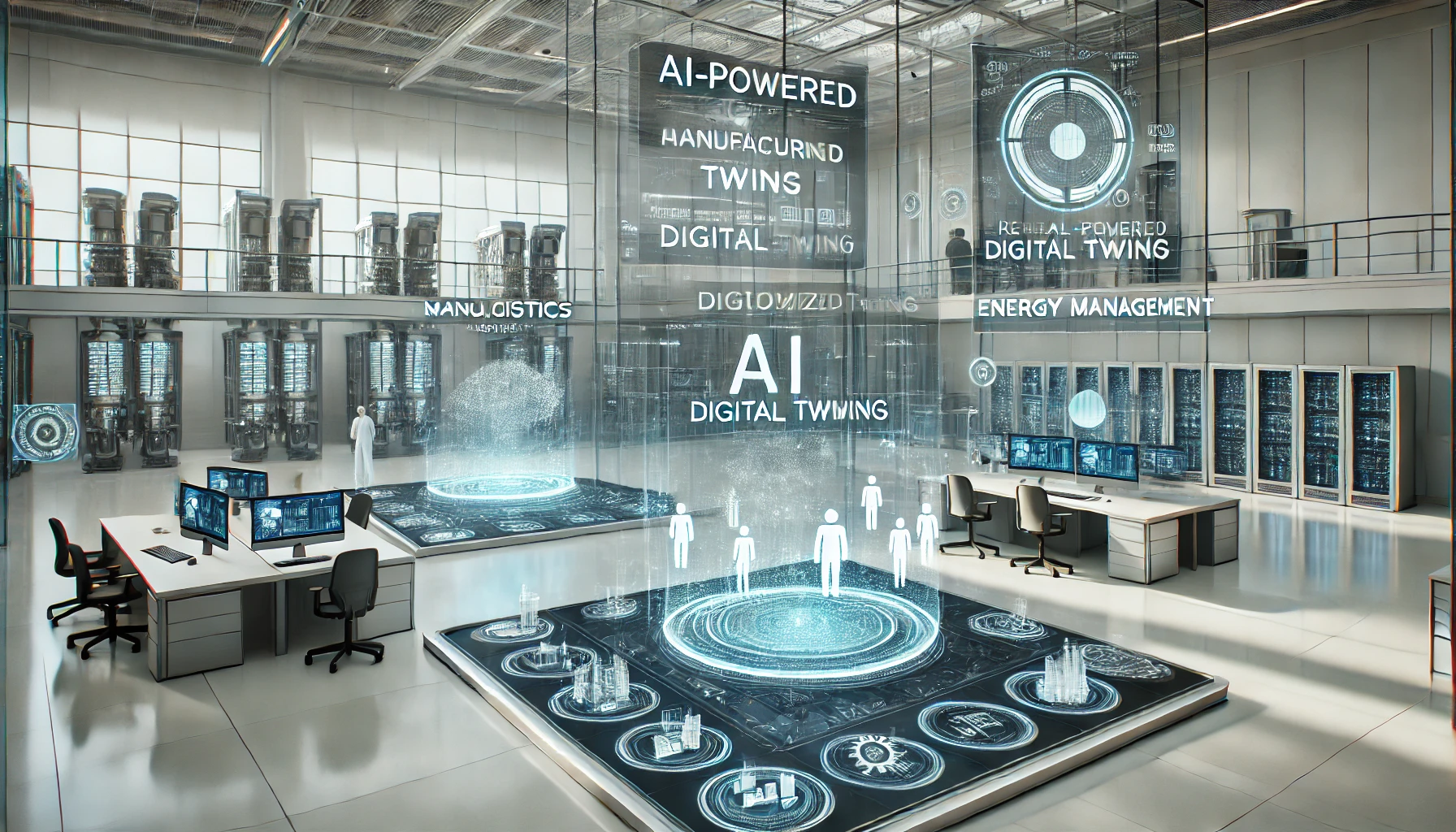
Conclusion
The trends explored in this blog highlight the transformative potential of AI automation across various sectors. By embracing these innovations, businesses can enhance operational efficiency, improve decision-making, and drive growth in an increasingly competitive landscape. As AI continues to evolve, staying informed and adapting to these advancements will be crucial for maintaining a competitive edge. Businesses are encouraged to explore AI automation services offered by specialised agencies to fully leverage these technologies and prepare for a future driven by intelligent automation.
Sources and Bibliography
OpenAI. (2023). GPT-5: Advancements in Natural Language Processing.
ResearchGate. (2023). Machine Learning Algorithms: Learning from Limited Data.
IEEE Xplore. (2023). Computer Vision Innovations and Applications.
Journal of Data Quality Management. (2023). Autonomous Data Quality Management Techniques.
International Journal of Digital Twins. (2023). AI-Powered Digital Twinning: Applications and Challenges.
By keeping abreast of these trends, businesses can strategically position themselves to harness the full potential of AI automation, ensuring not just survival but thriving in an evolving digital landscape.