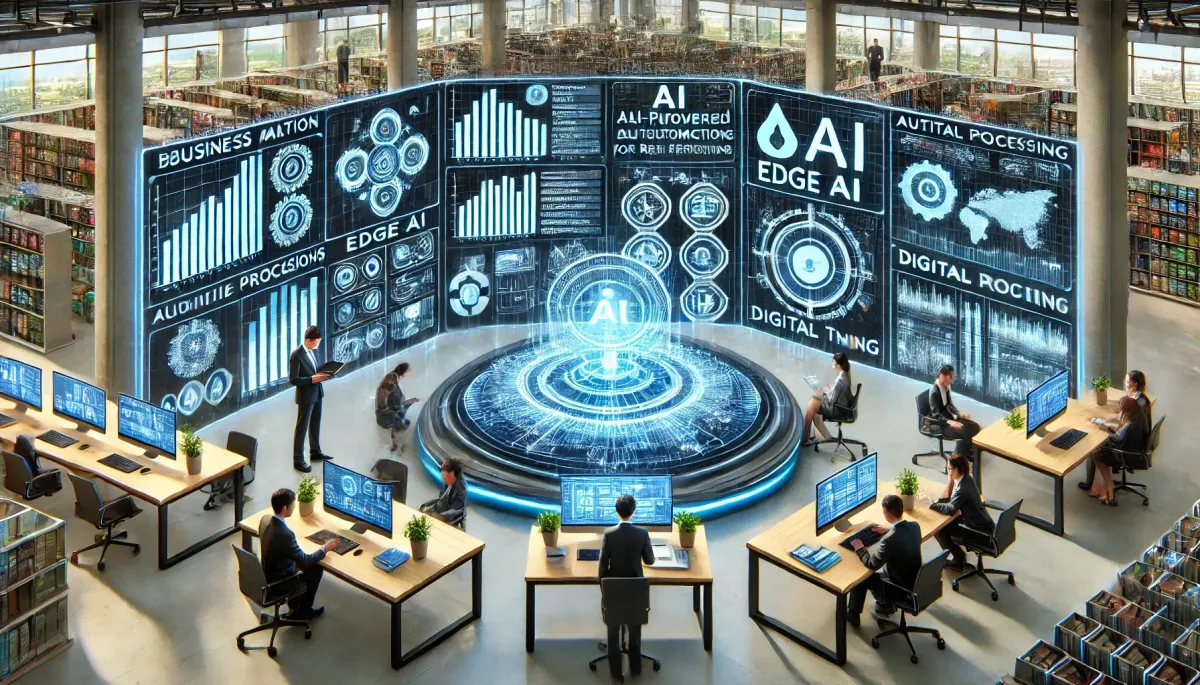
Weekly Insights: Transformative AI Automation Trends to Propel Your Business in 2025
Weekly Insights: Transformative AI Automation Trends to Propel Your Business in 2024
As businesses continue to embrace technological advancements, AI automation stands out as a pivotal force reshaping industries and enhancing operational efficiencies. In this blog, we’ll explore five emerging trends in AI automation for 2024, offering insights into innovations, challenges, and sector-specific applications that are fresh to the ongoing discourse in AI-powered workflows and web development automation.
1. AI-Driven Synthetic Data Generation
General Insights
AI-driven synthetic data generation is transforming how organisations handle data for AI model training. Unlike real-world data, which can be scarce or sensitive due to privacy concerns, synthetic data is artificially generated to mimic real-world datasets. This approach allows companies to circumvent data limitations while maintaining robust model training. Companies like Google and Amazon have recognised its potential, leading the charge in this innovative field.
Innovations
Recent technological advancements, particularly in generative adversarial networks (GANs) and variational autoencoders (VAEs), have significantly improved the quality of synthetic data. These models can generate datasets that are virtually indistinguishable from actual data, which is crucial for developing accurate AI models. This innovation opens up new possibilities for businesses to simulate a variety of scenarios without needing real-world data.
Challenges
Despite its advantages, synthetic data generation faces significant hurdles. Ensuring the diversity and representativeness of the generated data is critical to avoid introducing biases into AI models. Moreover, ethical considerations arise around the potential misuse of synthetic data, such as creating deepfakes or misleading content.
Case Studies and Applications
In healthcare, synthetic data has been a game changer. For instance, researchers at MIT used synthetic data to train AI models for detecting breast cancer from mammograms, achieving high accuracy levels. This demonstrates how synthetic data can enhance medical imaging applications, potentially saving lives by improving early detection rates.
Sector-Specific Insights
In finance, synthetic data is used to simulate market conditions, assisting in risk analysis and portfolio management. Retailers employ it to model customer behaviour, which enhances marketing strategies and customer segmentation. These applications highlight synthetic data's broad potential to transform industries by providing versatile and scalable data solutions.
2. Edge AI for Real-Time Processing
General Insights
Edge AI is revolutionising how data is processed by bringing AI capabilities closer to the source of data collection. This is achieved by deploying AI models at the edge of networks, such as on local devices or within IoT systems, which reduces latency and bandwidth usage. Major players like NVIDIA and Intel are heavily investing in this trend, recognising its capacity to facilitate real-time data processing.
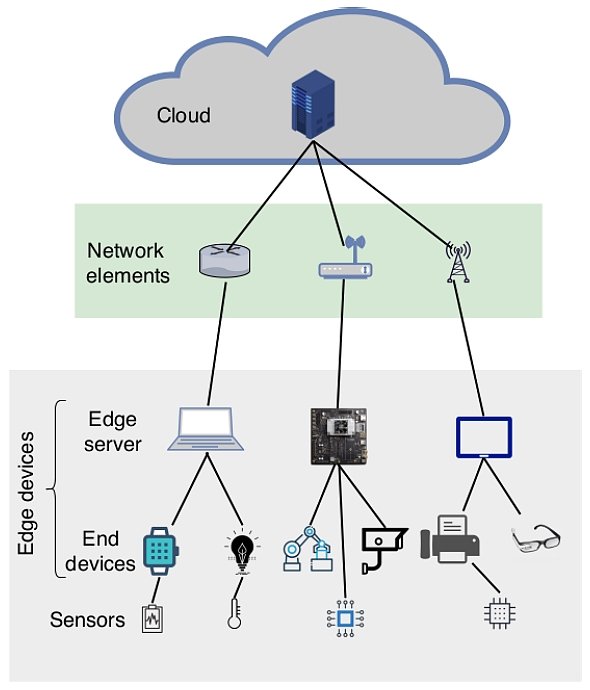
Innovations
The combination of edge computing with the explosion of IoT devices has made real-time data analysis feasible and efficient. This setup allows businesses to process information instantly, offering significant advantages in environments where speed and efficiency are crucial.
Challenges
One of the critical challenges with edge AI is ensuring the security and robustness of these systems. Since these models are often deployed in a distributed manner, they can be vulnerable to cyber threats. Additionally, bias in AI algorithms remains a concern, as these biases can lead to unequal outcomes across different demographics.
Case Studies and Applications
A prominent example is a real-time traffic monitoring system developed by Stanford researchers, which employs edge AI to detect traffic incidents and congestion with impressive accuracy. This showcases edge AI's potential in smart city initiatives, enhancing urban management and safety.
Sector-Specific Insights
In manufacturing, edge AI supports real-time monitoring of production processes, leading to increased operational efficiency. In transportation, it improves route optimisation and reduces congestion through real-time traffic management. In the energy sector, edge AI enables the real-time monitoring of energy systems, optimising resource allocation and consumption. These applications illustrate the transformative impact of edge AI across various industries.
3. AI-Driven Cybersecurity
General Insights
In the face of increasingly sophisticated cyber threats, AI-driven cybersecurity is becoming an essential line of defence for many organisations. Technologies that leverage AI for real-time threat detection and response are being developed by industry giants like IBM and Microsoft, recognising the need for enhanced security measures.
Innovations
Machine learning and deep learning advancements allow AI models to dynamically identify and mitigate cyber threats. These systems can process and analyse massive datasets, identifying anomalies that could indicate security breaches, thereby acting preemptively to protect valuable information.
Challenges
The main challenge lies in securing the AI models themselves, as they can become targets of adversarial attacks. Additionally, biases within AI systems could result in unfair targeting of specific groups, necessitating ongoing vigilance and refinement of these technologies.
Case Studies and Applications
At Carnegie Mellon, researchers developed an AI-driven cybersecurity system that successfully detected and responded to cyber attacks on a major financial institution. This case underscores the significant potential of AI-driven cybersecurity to protect sensitive data and maintain the integrity of digital platforms.
Sector-Specific Insights
In finance, AI-driven cybersecurity assists in detecting fraud and responding to breaches, thereby enhancing risk management. Healthcare organisations use these systems to protect patient data, which is critical for maintaining trust and compliance with regulations. In the retail sector, AI-driven solutions safeguard customer data, helping to preserve consumer trust and confidence in digital transactions.
4. AI-Driven Content Generation
General Insights
AI-driven content generation is reshaping how organisations create and distribute content across various sectors. Companies like Google and Amazon are investing heavily in this trend, recognising its potential to streamline content production and enhance audience engagement through automated processes.
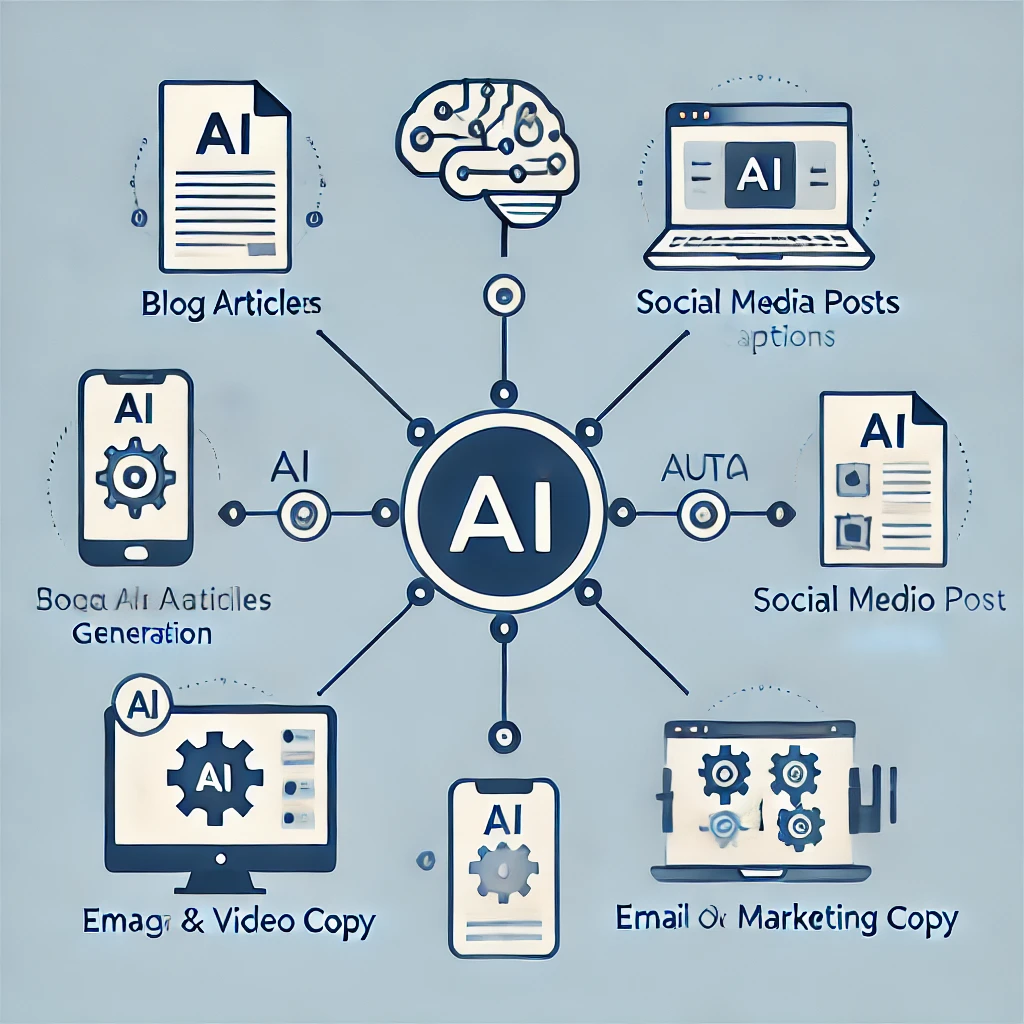
Innovations
Advancements in natural language processing (NLP) and computer vision enable AI to generate high-quality text, images, and videos. These tools allow organisations to tailor content creation to meet specific audience needs, significantly reducing the time and effort traditionally required in content development.
Challenges
Ensuring the diversity and quality of AI-generated content remains a challenge, along with ethical concerns about misinformation and the potential for creating deepfakes. These issues necessitate careful oversight and governance to maintain content integrity.
Case Studies and Applications
At UC Berkeley, researchers developed an AI system that can generate accurate text summaries of news articles. This innovation demonstrates AI's capability to enhance media applications, offering a glimpse into future possibilities for automating content creation.
Sector-Specific Insights
In the media sector, AI is used to produce engaging content, streamlining the production process and improving audience interaction. In marketing, AI generates personalised materials, enhancing customer outreach and increasing conversion rates. In education, AI-driven content creation is used to develop online courses and textbooks, improving accessibility and broadening educational reach.
5. AI-Driven Digital Twinning
General Insights
AI-driven digital twinning involves creating virtual replicas of physical systems, allowing for enhanced simulation and analysis. This trend is gaining momentum as companies like Siemens and GE invest in digital twinning technologies, recognising its potential to optimise processes and resources.
Innovations
Recent advancements in machine learning and computer vision have enabled the creation of accurate digital twins, which allow businesses to simulate real-world scenarios and optimise operations without physical trial and error.
Challenges
The primary challenge lies in ensuring the accuracy of digital twins, as they must precisely replicate real-world conditions to be effective. Additionally, the data used to create these models must be free from bias to prevent skewed simulations and results.
Case Studies and Applications
At MIT, researchers successfully developed a digital twin of a major manufacturing facility, showcasing how digital twins can optimise operational processes. This application highlights the potential of digital twinning to transform industries by providing deeper insights into system performance and optimisation.
Sector-Specific Insights
In manufacturing, digital twins simulate production processes, enabling predictive maintenance and efficiency improvements. In the energy sector, they model energy systems to optimise resource management and reduce waste. In transportation, digital twins enhance the simulation of systems, improving logistics and urban planning.
Conclusion
The landscape of AI automation is rapidly evolving, presenting new trends that promise to boost operational efficiencies and drive innovation across various sectors. By understanding and leveraging these advancements, businesses can position themselves at the forefront of the AI revolution. Companies are encouraged to explore these trends to enhance their operations and consider partnering with expert agencies to implement these technologies effectively.