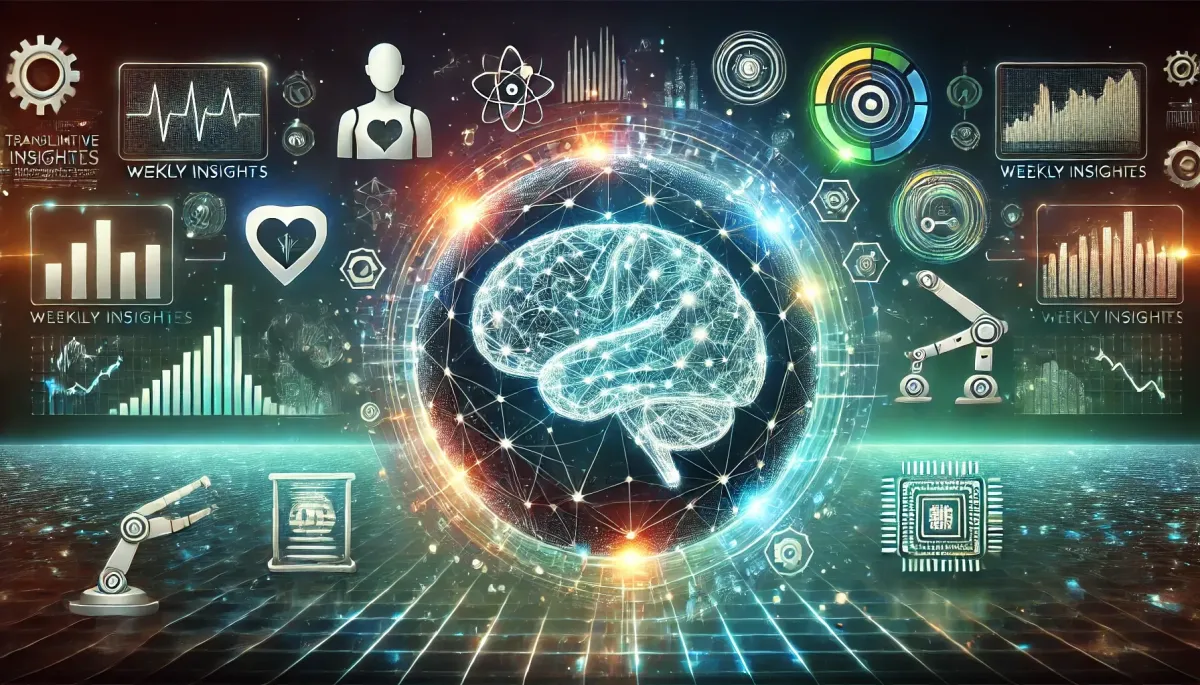
Weekly Insights: Transformative AI Automation Trends to Watch in 2024
As artificial intelligence (AI) and automation continue to evolve, businesses must stay informed about emerging trends to maintain their competitive edge. This blog explores five key areas in AI automation that are set to reshape industries in 2024, offering insights into innovations, challenges, and practical applications. By understanding these trends, businesses can harness AI's potential to drive innovation and efficiency in an ever-evolving digital landscape.
1. New AI Models and Engines
Innovations
The introduction of advanced AI models like Llama 2 and the anticipated GPT-5 is revolutionising natural language processing (NLP). These models enhance language understanding and conversational capabilities, making interactions between humans and machines more intuitive. Think of these models as the upgraded brains of digital assistants, capable of processing vast amounts of information to provide insightful and context-aware responses.
Applications
In healthcare, for instance, Llama 2 is improving clinical decision-making and patient engagement by offering precise and timely information. This model is akin to having a highly knowledgeable assistant who never forgets any detail and is always ready to provide insights—whether it's helping doctors diagnose conditions or suggesting treatment plans based on historical data.
Challenges
Despite their capabilities, these models raise concerns about data privacy, security, and the potential for biased outputs. Businesses must tread carefully, ensuring that their AI deployments adhere to ethical guidelines and data protection laws. It's like navigating a new technological landscape where the journey to success is as important as the destination.
Case Studies
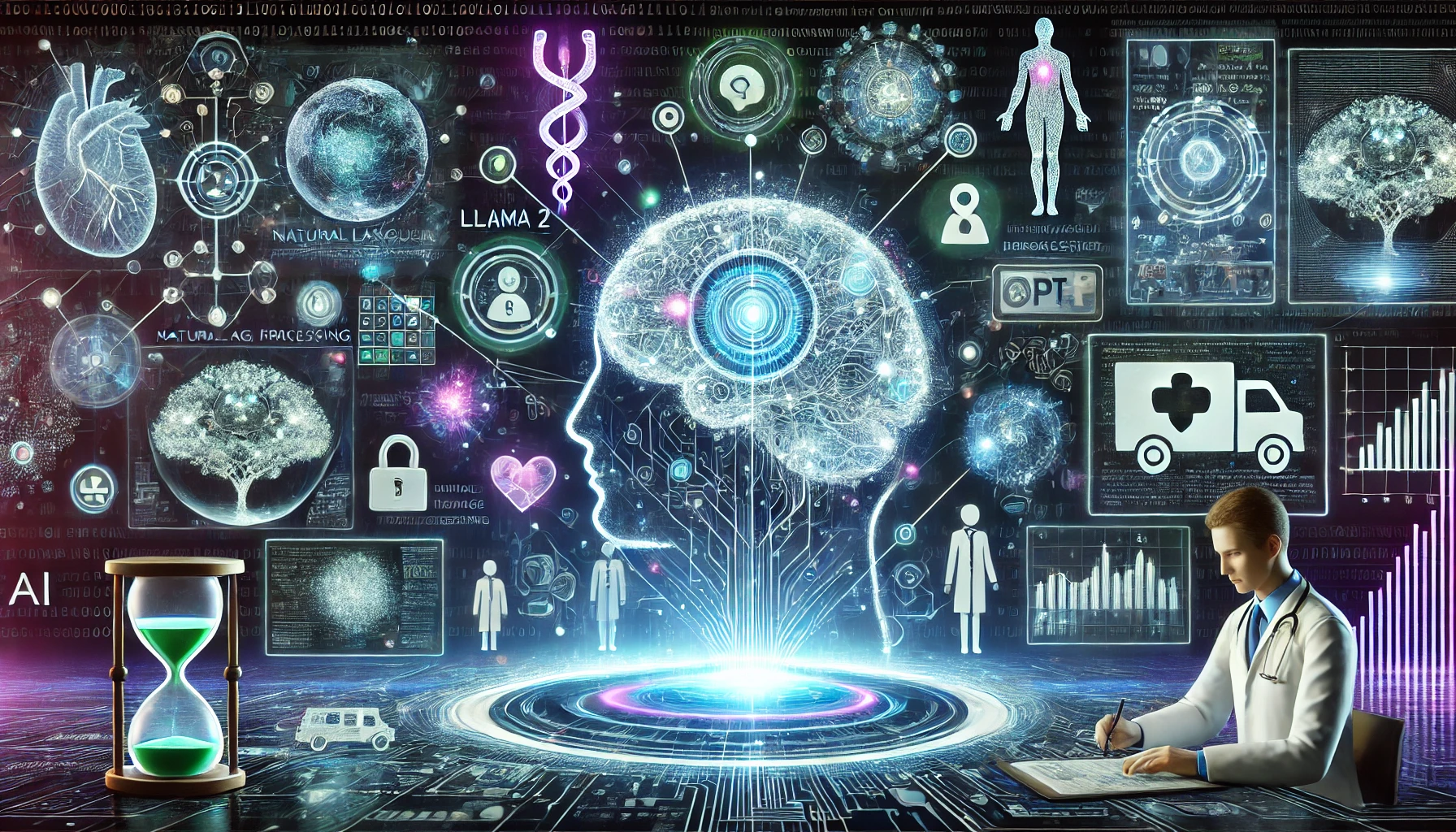
The University of California, San Francisco, showcased Llama 2's potential by implementing it in intensive care units to analyse patient data and offer actionable insights. This case highlights how cutting-edge AI can drive better patient outcomes and streamline complex healthcare processes.
2. Machine Learning Algorithms for Dynamic Environments
Innovations
Recent advancements focus on machine learning algorithms that adapt to dynamic data environments. These algorithms learn continuously, adjusting their models as new data comes in, which is crucial for fast-paced industries. Imagine them as a team of analysts who never sleep, constantly updating their insights as new information arises.
Applications
In the finance sector, these adaptive algorithms enhance risk management and fraud detection by swiftly identifying and responding to new transaction patterns. For example, if a new type of fraudulent transaction is detected, the system can quickly adapt—much like a vigilant security system that recalibrates in real-time to new threats.
Challenges
The key challenge is ensuring the robustness and reliability of these algorithms. They must be tested rigorously to perform well under diverse conditions without introducing biases. This is akin to stress-testing a bridge to ensure it holds up under various weather conditions and loads.
Case Studies
The Bank of England has effectively utilised these algorithms for portfolio optimisation and risk assessment, demonstrating significant improvements in efficiency and reliability. This success story illustrates how adaptive machine learning can be a game-changer in data-driven industries.
3. Advancements in Computer Vision
Innovations
Computer vision technology is advancing rapidly, particularly in visual data analytics. New models improve image recognition and object detection, enhancing their capabilities across various sectors. Think of computer vision as the digital eye that can see and interpret the world more accurately than ever before.
Applications
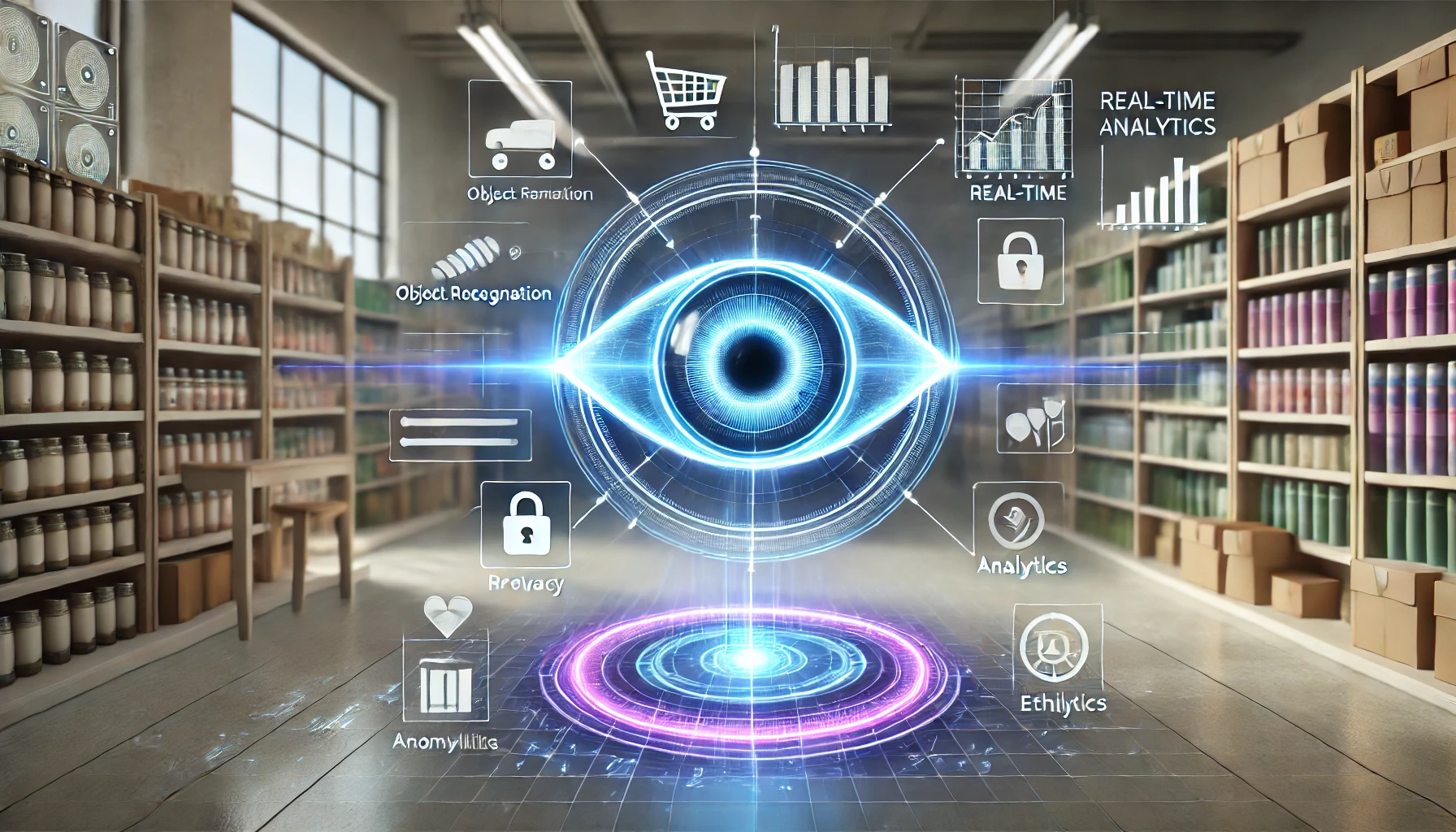
In retail, computer vision is revolutionising inventory management and personalising marketing strategies by analysing customer behaviour and preferences. It's like having a smart assistant who can predict what customers want even before they ask, ensuring shelves are stocked and promotions are timely.
Challenges
Deploying these systems raises ethical issues, especially concerning privacy and data security. Businesses must be transparent about data collection practices and comply with regulations, akin to balancing the benefits of surveillance with privacy rights.
Case Studies
Walmart's use of computer vision for inventory management resulted in fewer stockouts and increased customer satisfaction, showcasing the real-world impact of integrating advanced visual analytics.
4. Autonomous Data Quality Management
Innovations
Autonomous data quality management is becoming essential as organisations strive for high data integrity. AI-driven systems can now automatically detect and correct discrepancies, ensuring operations are based on reliable data. Imagine these systems as digital custodians that maintain the health and cleanliness of your data environment.
Applications
In finance and healthcare, where accuracy is critical, these solutions enhance decision-making by ensuring data reliability. For example, in healthcare, accurate patient data is crucial for effective treatment—much like how a clean and well-organised pantry is essential for efficient meal preparation.
Challenges
Implementing these systems can be complex, requiring integration with existing infrastructure and ensuring AI systems are well-trained to identify data issues. This is akin to integrating a new organ into a living body, ensuring it functions harmoniously with the rest.
Case Studies
General Electric has leveraged autonomous data quality management to enhance product quality and reduce manufacturing defects, illustrating the operational benefits of maintaining high data integrity.
5. LLM Advancements for Training Other AI Models
Innovations
Large language models (LLMs) are proving invaluable in training other AI models more efficiently. They streamline the process, reducing the time and resources required for training new models. Consider them as experienced mentors for new AI models, imparting knowledge quickly and effectively.
Applications
In sectors like healthcare and finance, where developing predictive models is resource-intensive, LLMs expedite training and improve performance. It's like using a master chef to train junior chefs, ensuring they learn quickly and accurately.
Challenges
The main challenge is ensuring LLMs are finely tuned for specific applications, which requires expertise and resources for adaptation. This is similar to customising a suit to fit perfectly, ensuring it complements the wearer’s needs.
Case Studies
Research published in the Journal of Machine Learning Research demonstrated LLMs' efficacy in training predictive healthcare models, significantly reducing training times while enhancing accuracy.
Conclusion
AI and automation are at the forefront of innovation, with trends like advanced AI models, adaptive machine learning, computer vision, autonomous data management, and LLM advancements leading the charge. Businesses that embrace these technologies can enhance operational efficiency and maintain a competitive edge. Staying informed and adaptable is crucial as the landscape continues to evolve. For those looking to integrate these advancements, partnering with a knowledgeable AI and web development agency can provide the expertise needed to successfully navigate this technological transformation.