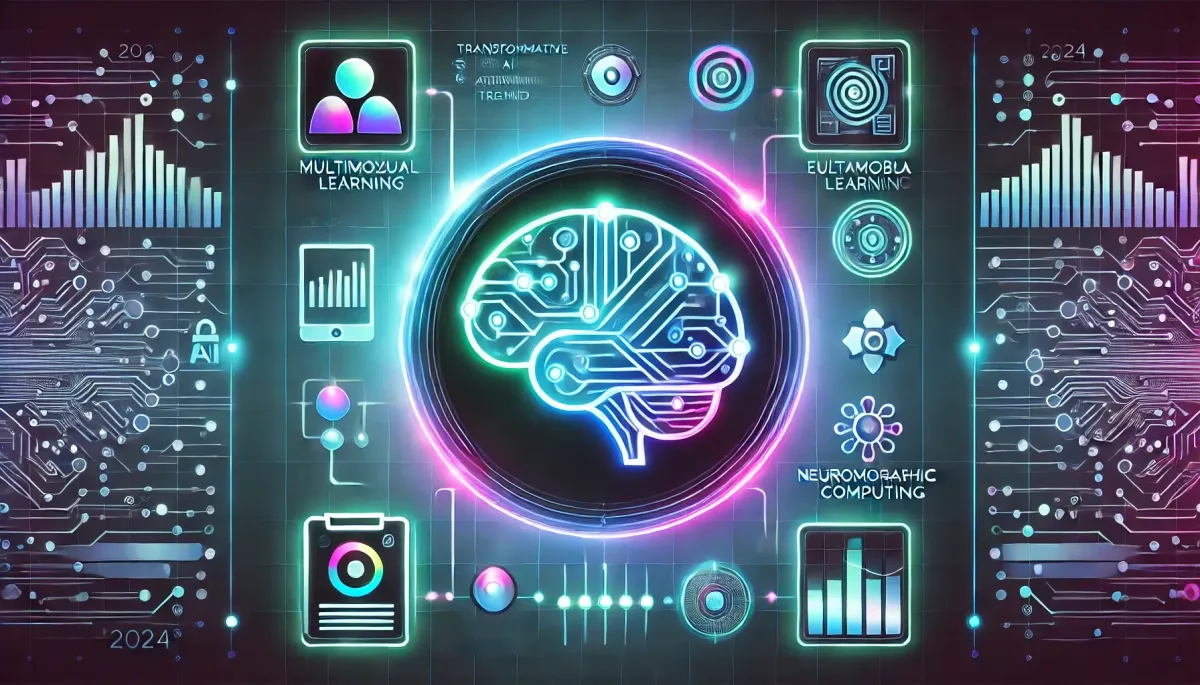
Weekly Insights: Transformative AI Automation Trends to Watch Now
As we journey deeper into 2024, the realm of AI automation is rapidly evolving, presenting fresh opportunities and challenges for businesses across various industries. This blog explores five innovative trends in AI automation that are set to transform sectors ranging from healthcare to finance, offering new insights and practical applications for businesses eager to harness these technologies.
Advancements in Multimodal Learning for AI Automation
General Insights and Innovations
Multimodal learning represents a cutting-edge area in AI automation tools, where the integration of diverse data types—such as text, images, and audio—enhances the understanding and predictive capabilities of AI models. This approach leverages the power of deep learning architectures like the Multimodal Transformer models, which excel in complex tasks such as visual question answering and sentiment analysis by processing and interpreting data from multiple sources simultaneously.
Challenges and Case Studies
The main challenge of multimodal learning lies in integrating various data formats into a cohesive model, which requires extensive labelled datasets for training. Despite these challenges, the potential benefits are significant. For example, in healthcare, multimodal learning is revolutionising diagnostic processes by combining imaging data, such as MRI scans, with patient histories to improve cancer detection. A study in Nature Medicine highlighted this by enhancing diagnostic accuracy and treatment planning through such integrative approaches.
Sector-Specific Insights
In the finance sector, this technology can merge financial reports with real-time news articles, providing a comprehensive risk analysis that informs better decision-making. This holistic approach allows businesses to stay ahead in the dynamic market landscape, identifying trends and threats more effectively than traditional methods.
Explainable Planning in AI Decision-Making
General Insights and Innovations
Explainable planning focuses on developing AI systems capable of elucidating their decision-making processes. This transparency is crucial as AI becomes embedded in critical applications, necessitating clear and accountable decision frameworks. Innovations like model-agnostic techniques enable systems to provide stakeholders with understandable insights into AI-driven decisions.
Challenges and Case Studies
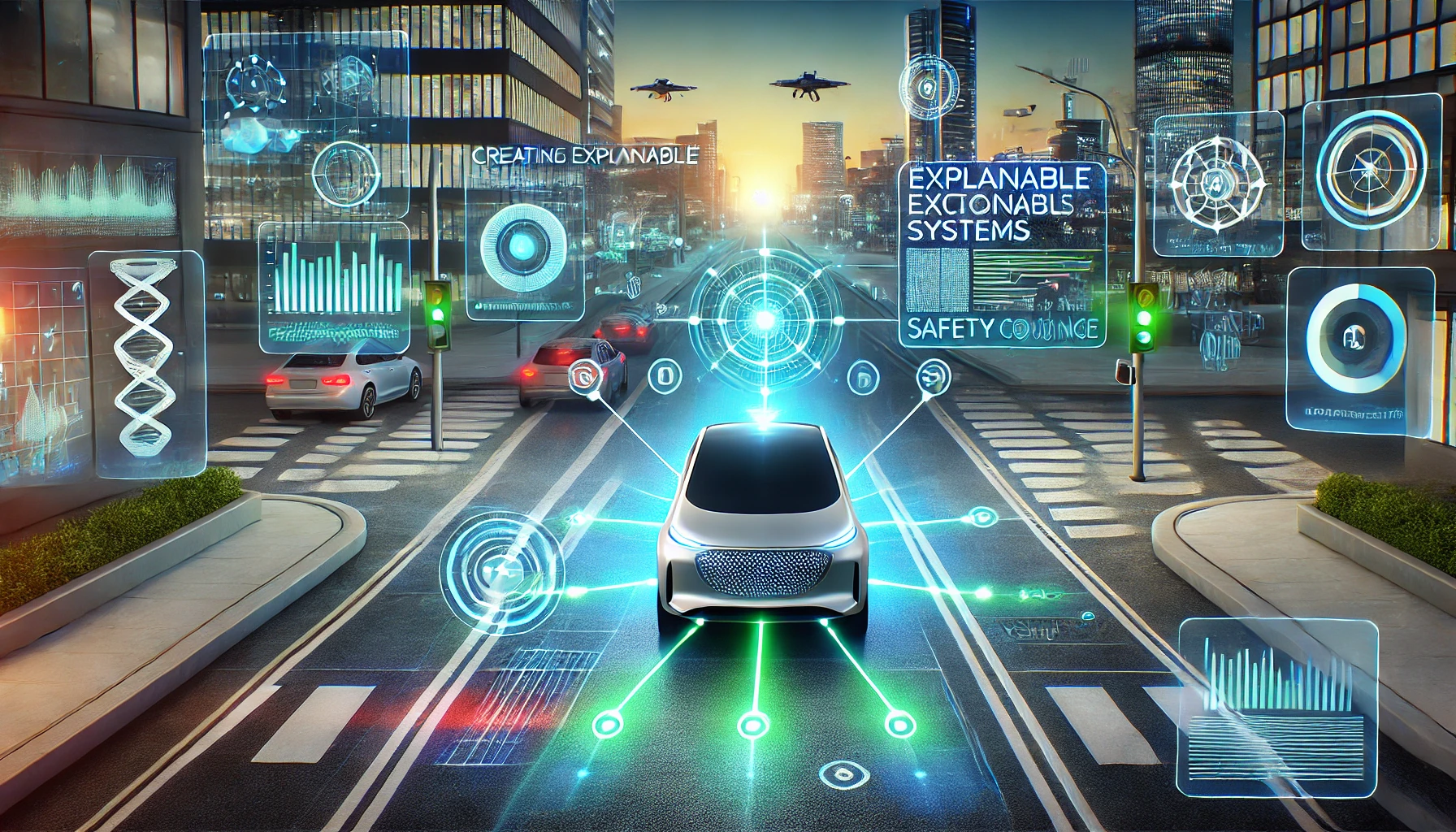
Despite the advancements, crafting clear and concise explanations from AI systems remains complex, particularly in intricate decision-making scenarios. A notable application is in autonomous vehicles, where AI systems must articulate navigation choices for safety and compliance reasons. Research in IEEE Transactions on Intelligent Transportation Systems demonstrated real-time explainability in autonomous driving, enhancing user trust and regulatory adherence.
Sector-Specific Insights
In healthcare, explainable AI improves patient engagement by providing understandable explanations for diagnoses and treatment plans, fostering trust and adherence. This transparency can be pivotal for both medical professionals and patients, ensuring AI-driven insights are actionable and credible.
Autonomous Data Quality Management for Web Development
General Insights and Innovations
As businesses increasingly rely on data for decision-making, maintaining high data quality is essential. Autonomous data quality management utilises AI to detect and rectify data inconsistencies, ensuring data integrity. Recent innovations in data validation and cleansing streamline the maintenance of quality data, which is crucial for accuracy in web development automation projects.
Challenges and Case Studies
The challenge lies in achieving high detection accuracy and correcting errors without human intervention. However, a study published in IEEE Transactions on Knowledge and Data Engineering highlighted the successful deployment of these systems in web applications, significantly reducing errors and improving data reliability.
Sector-Specific Insights
In finance, autonomous data quality management enhances the precision of risk assessments and credit scoring, which are crucial for informed decision-making. For web developers, it means building applications with reliable data inputs, ensuring user trust and satisfaction.
Neuromorphic Computing for Efficient Data Analysis
General Insights and Innovations
Neuromorphic computing seeks to replicate the neural architecture of the human brain, aiming to process information efficiently and effectively. This approach, utilising architectures such as spiking neural networks, is particularly promising for real-time data analysis, offering speed and accuracy enhancements.
Challenges and Case Studies
Developing systems that mimic brain functions poses significant challenges, requiring advanced neuroscientific understanding. Despite these hurdles, research in Nature Communications demonstrated the potential of neuromorphic computing in high-accuracy image classification, underscoring its applicability in real-time scenarios.
Sector-Specific Insights
In healthcare, neuromorphic computing can transform medical imaging analysis, leading to quicker and more accurate diagnoses. This efficiency can drastically improve patient outcomes by facilitating prompt and precise medical interventions.
Human-AI Collaboration for Enhanced Decision-Making
General Insights and Innovations
The trend of human-AI collaboration focuses on creating AI systems that complement human decision-making, blending computational precision with human intuition. Techniques such as human-in-the-loop systems are being developed to enable effective collaboration, crucial in sectors like healthcare and finance.
Challenges and Case Studies
The integration of AI and human workflows presents challenges in understanding human decision processes and the resource demands of training collaborative systems. Nevertheless, a study in IEEE Transactions on Human-Machine Systems explored AI systems that assist healthcare professionals in improving diagnostic accuracy, highlighting the benefits of such collaboration.
Sector-Specific Insights
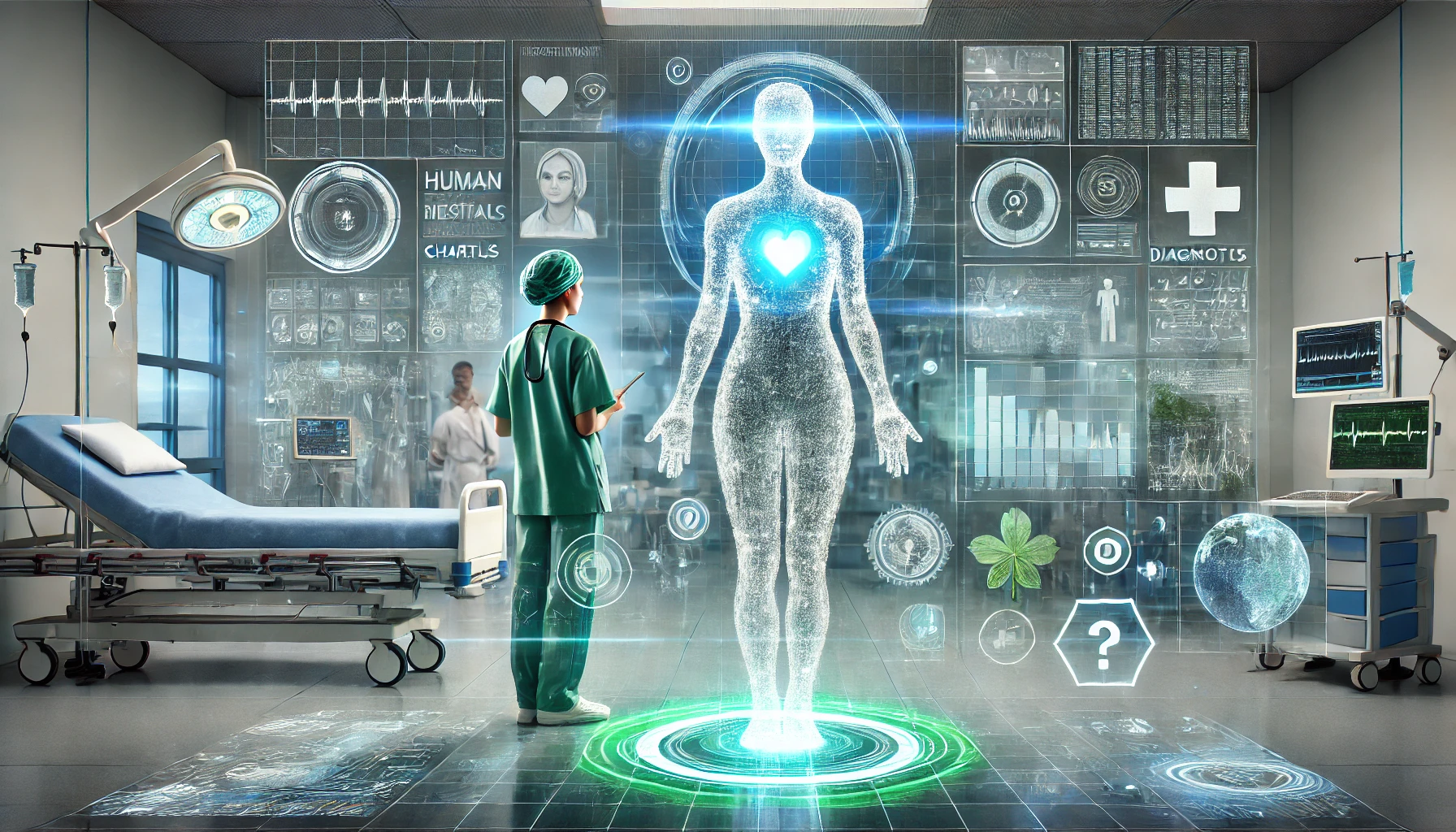
In healthcare, this collaboration reduces diagnostic errors and enhances treatment planning, ultimately improving patient outcomes. For businesses, integrating human insight with AI analysis can lead to more nuanced and effective decision-making processes, providing a competitive edge.
Conclusion
The trends explored in this blog illustrate the dynamic evolution of AI automation in 2024. By embracing advancements in multimodal learning, explainable planning, autonomous data quality management, neuromorphic computing, and human-AI collaboration, businesses can leverage AI's full potential to drive innovation and improve decision-making. As these technologies continue to develop, organisations must adapt and integrate them to enhance efficiency and remain competitive. For those ready to embark on this transformative journey, exploring AI automation platforms like Make.com and GoHighLevel will be crucial for staying at the forefront of industry innovation.